Introduction: The Rising Need for AI in Cybersecurity Due to Increasingly Sophisticated Threats
In today’s hyper-connected world, cybersecurity has never been more critical. With businesses, governments, and individuals relying on digital infrastructure for everyday operations, the threats facing them have become increasingly sophisticated, persistent, and dangerous. Cybercriminals are no longer just using simple tactics but have developed highly advanced methods of breaching systems, from phishing scams to ransomware attacks, and even state-sponsored cyber espionage. As a result, traditional cybersecurity methods, which often rely on predefined rules and human oversight, are struggling to keep pace with the evolving tactics of cyber attackers.
This is where artificial intelligence (AI) comes into play. AI, particularly machine learning (ML) and deep learning, has the potential to transform cybersecurity by automating threat detection, improving response times, and even predicting new threats before they happen. By leveraging the power of AI to analyze vast amounts of data, detect patterns, and respond in real-time, businesses and individuals can stay one step ahead of cybercriminals.
In this article, we will explore how AI is being used to defend against cybersecurity threats, the benefits of machine learning in malware analysis, the critical role of human-AI collaboration, and the challenges and risks of relying on AI in cybersecurity.
AI for Threat Detection: How AI is Helping Detect and Mitigate Cyber-Attacks in Real-Time
One of the most exciting applications of AI in cybersecurity is its ability to detect and mitigate cyber-attacks in real-time. Traditional cybersecurity systems, such as firewalls and antivirus software, rely on predefined signatures and patterns to recognize known threats. While these tools are effective against known malware, they fall short when confronted with new, unknown, or highly sophisticated attacks. This is where AI-powered threat detection comes into play.
- Real-Time Threat Detection: AI-based systems can analyze network traffic, system logs, and other data sources to identify suspicious activity and detect anomalies that could indicate a cyber-attack. By using machine learning algorithms, AI can recognize patterns of behavior that deviate from the norm and flag them as potential threats. These AI systems can then automatically trigger responses, such as isolating compromised systems or blocking malicious IP addresses, to mitigate the impact of an attack before it can cause significant damage.
- Behavioral Analysis: Unlike traditional systems that rely on known signatures, AI-powered systems focus on behavioral analysis. These systems learn what normal, healthy activity looks like within a network and can identify irregularities that might indicate malicious behavior. This means that AI can detect new, previously unseen threats based on their behavior rather than their specific signature. For example, AI can spot abnormal data exfiltration or sudden spikes in network traffic, both of which could suggest a hacking attempt or a distributed denial-of-service (DDoS) attack.
- Reducing False Positives: One of the challenges with traditional cybersecurity systems is the high volume of false positives—alerts about threats that turn out to be benign. This can overwhelm security teams and lead to alert fatigue. AI helps reduce these false alarms by continually learning from past events and fine-tuning its detection models. This results in more accurate threat detection and allows security teams to focus on real threats rather than spending time sifting through countless alerts.
Machine Learning in Malware Analysis: Enhancing Malware Detection Through AI
Malware, including viruses, worms, and ransomware, remains one of the most persistent cybersecurity threats. Traditional antivirus software often relies on known virus definitions or signatures to identify malware, but cybercriminals frequently develop new strains of malware that go undetected by these methods. AI, specifically machine learning, has the ability to revolutionize malware detection by learning how to identify malicious patterns and behaviors rather than just known threats.
- Static and Dynamic Analysis: Machine learning can be applied to both static and dynamic analysis of software. Static analysis involves examining the code of a file without executing it, while dynamic analysis involves running the file in a controlled environment to observe its behavior. By analyzing both the code and behavior of software, machine learning algorithms can more accurately predict whether a file is malicious. This allows AI to identify new and previously unknown types of malware that have not been encountered before.
- Signature-Free Malware Detection: Machine learning models do not require predefined signatures to identify threats. Instead, they learn from vast datasets of legitimate and malicious files, gaining an understanding of what constitutes harmful behavior. These models can then apply this knowledge to detect novel malware strains, even if the specific code or behavior has never been seen before. This ability to detect new types of malware is critical in the face of rapidly evolving cyber threats.
- Automated Malware Analysis: Traditional malware analysis often involves manual investigation, which can be time-consuming and resource-intensive. AI can automate much of this process by quickly analyzing large volumes of files and providing actionable insights to security teams. This significantly speeds up the detection and response time, reducing the window of opportunity for cybercriminals to exploit vulnerabilities.
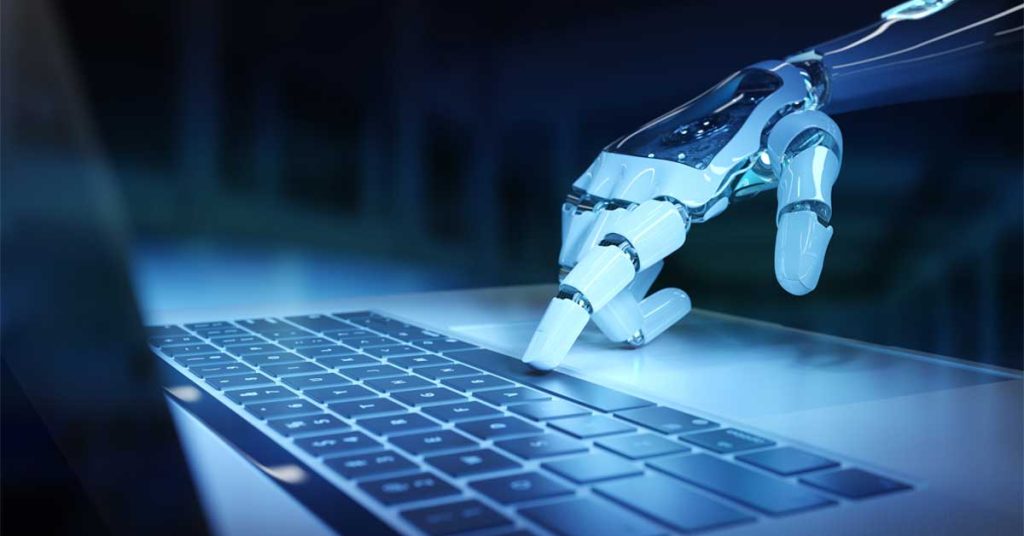
AI and Human Collaboration: Combining AI with Human Oversight for Better Security
While AI is a powerful tool for enhancing cybersecurity, it’s important to recognize that it is not a perfect solution on its own. Human oversight remains essential in ensuring that AI-driven security systems function effectively and responsibly. The ideal approach is a human-AI collaboration, where AI performs the heavy lifting of data analysis and threat detection, while human experts provide context, judgment, and decision-making.
- Human Judgment in Complex Situations: AI is excellent at processing large volumes of data and identifying patterns, but it can struggle with complex, ambiguous situations that require human intuition. Security experts can step in to provide insights and validate AI findings, particularly in cases where the context of a potential threat is nuanced. For example, AI may flag a suspicious login attempt, but a human may recognize it as a legitimate action by an employee working from a different location.
- Training AI Models: One of the critical roles that humans play in the AI process is training the machine learning models. Human security analysts provide labeled data—such as examples of known malware, phishing emails, and other threats—that the AI can use to learn. Over time, as AI continues to process more data, it becomes increasingly effective at identifying emerging threats.
- Ethical and Legal Oversight: AI systems may sometimes encounter ethical dilemmas or raise legal concerns, such as the potential for privacy violations. Human oversight is essential to ensure that AI-powered cybersecurity tools are used responsibly, adhering to legal and ethical guidelines. Security teams must ensure that AI algorithms do not inadvertently violate user privacy or make discriminatory decisions.
Challenges and Risks: The Potential for AI to Be Used Against Cybersecurity Efforts
Despite the many benefits of AI in cybersecurity, there are also challenges and risks that must be considered. Cybercriminals can potentially leverage AI for malicious purposes, and AI systems themselves may be vulnerable to exploitation.
- AI-Powered Cyberattacks: Cybercriminals are becoming increasingly savvy and are already using AI to launch sophisticated attacks. For example, AI-driven phishing attacks can be more targeted and convincing, using machine learning to mimic a trusted sender’s writing style or anticipate the best time to send a malicious email. AI could also be used to create self-learning malware that adapts to evade detection.
- Adversarial AI: Adversarial AI refers to attacks that manipulate AI models by providing them with misleading or biased data. In the context of cybersecurity, attackers could feed false data into an AI-powered security system to cause it to miss an attack or make incorrect judgments. Protecting AI systems from adversarial manipulation is a growing area of research in the cybersecurity field.
- Bias in AI Models: Machine learning models are only as good as the data they are trained on. If the data used to train an AI system contains biases, the model may produce biased results, leading to inaccurate threat detection. It’s essential to ensure that the data used to train AI systems is diverse, representative, and free from inherent biases.
Conclusion: How AI Will Continue to Play a Key Role in Cybersecurity Defense
As cyber threats become more advanced, AI will continue to be a crucial tool in the fight against cybercrime. AI’s ability to detect threats in real-time, analyze malware, and predict new attack vectors makes it an invaluable asset for security teams. However, for AI to be truly effective, it must be used in conjunction with human expertise, ethical guidelines, and continuous oversight.
The future of cybersecurity will undoubtedly be defined by the collaboration between human ingenuity and AI’s capabilities, leading to stronger, faster, and more adaptive defense systems. As AI technology continues to evolve, it will not only help protect against current threats but also provide the tools to anticipate and prevent the cyber-attacks of tomorrow.
Discussion about this post