Introduction: The Use of AI in Transforming Drug Discovery
The pharmaceutical industry has always been at the cutting edge of medical advancements, yet the process of drug discovery remains notoriously slow and expensive. Traditional drug development often involves years of research, vast amounts of data, and millions—sometimes billions—of dollars. However, with the rise of artificial intelligence (AI), the landscape of drug discovery is undergoing a transformative shift. AI models, powered by machine learning (ML) and deep learning (DL), have become invaluable tools in predicting the effectiveness of drugs, identifying new therapeutic candidates, and optimizing existing treatments.
By using vast datasets to uncover patterns that were previously inaccessible to human researchers, AI offers a more efficient and cost-effective way to navigate the complex and lengthy drug discovery process. The ability to simulate and predict biological interactions and molecular behaviors can accelerate the identification of promising drug candidates while minimizing the risks and costs associated with human trials. This revolution in pharmaceutical research is not just speeding up the time-to-market for new treatments but also expanding the range of possibilities for diseases previously considered difficult or impossible to treat.
This article delves into the role of AI in drug discovery, exploring how it works, showcasing successful case studies, and examining its potential for personalized medicine. We will also look at the challenges and ethical considerations that arise when relying on AI models in such a high-stakes field.
How AI Models Work: Machine Learning and Data Analysis for Predicting Drug Efficacy
At the core of AI-powered drug discovery are machine learning and data analytics. These technologies allow computers to learn from vast amounts of data, improving their predictive capabilities without explicit programming. In the context of drug discovery, these models analyze data from a variety of sources, including:
- Chemical Databases: AI can process vast chemical libraries to predict how different compounds might interact with biological systems. This is especially useful in identifying promising molecules that can be further developed into drugs.
- Genomic Data: Advances in genomics have produced massive datasets on human DNA, enabling AI to predict which genetic variations might influence how a person responds to a particular drug. These insights can help design drugs that are tailored to an individual’s genetic makeup, improving both efficacy and safety.
- Clinical Trials Data: AI can also sift through data from past clinical trials to identify patterns that may indicate how certain drugs behave in different populations, helping researchers avoid costly mistakes and refine treatment strategies.
- Biological Pathways: AI can analyze complex biological systems to understand how diseases progress and identify potential drug targets. Machine learning algorithms can identify molecular targets that may be involved in disease mechanisms, enabling more focused drug design.
AI models do this by recognizing patterns, making connections, and predicting outcomes based on historical data. For example, deep learning techniques use neural networks to analyze molecular structures and predict their potential interactions with proteins or genes, which could lead to the development of new drugs or the repurposing of existing ones.
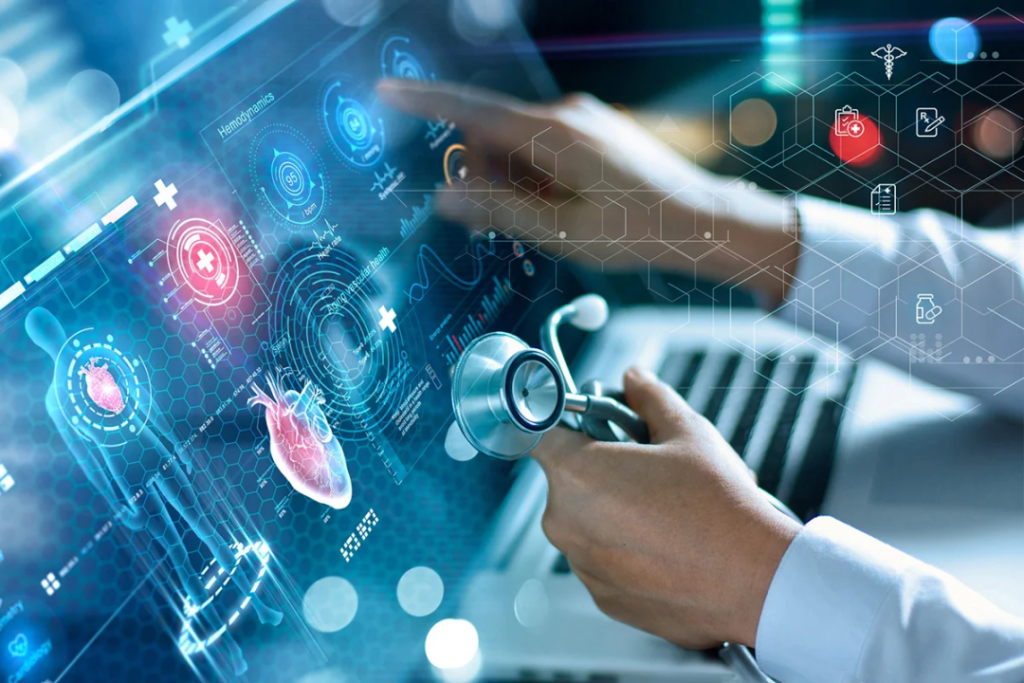
Case Studies: Successful AI-Driven Drug Discoveries in Recent Years
The integration of AI in drug discovery has already yielded some promising results. Several notable AI-driven breakthroughs have shown the potential of machine learning in accelerating the development of new treatments:
- Insilico Medicine’s Drug Discovery for Fibrosis: In 2020, the AI company Insilico Medicine used deep learning to identify a potential drug for pulmonary fibrosis, a condition with no approved treatments. Within just 46 days, AI algorithms helped researchers design a novel compound, which was later successfully tested in the lab. This represents a breakthrough in the use of AI to design drug candidates from scratch, a process that traditionally takes much longer.
- BenevolentAI and COVID-19: During the COVID-19 pandemic, BenevolentAI, an AI-focused drug discovery company, leveraged AI to identify a promising drug candidate, Baricitinib, for the treatment of COVID-19. By analyzing existing databases and predicting how the drug could inhibit the virus’s replication, BenevolentAI was able to repurpose an existing medication to treat the disease, speeding up the response to the pandemic.
- Atomwise and Ebola: Atomwise, a company specializing in AI-powered drug discovery, used machine learning to screen over 10 million compounds to identify potential treatments for Ebola. Their AI algorithm was able to predict how various molecules could bind to the Ebola virus, ultimately leading to the identification of promising drug candidates. Atomwise’s work in AI-driven screening has extended to several other diseases, including Zika virus and multiple sclerosis.
These examples highlight how AI can significantly speed up the discovery process, leading to faster identification of therapeutic candidates and more efficient repurposing of existing drugs.
AI in Personalized Medicine: Tailoring Treatments Using AI Insights from Patient Data
One of the most exciting applications of AI in drug discovery is its potential to revolutionize personalized medicine. By using AI to analyze patient-specific data—such as genetic information, medical history, and lifestyle factors—researchers can create treatments tailored to the individual, rather than relying on a one-size-fits-all approach.
- Pharmacogenomics: AI can help integrate pharmacogenomic data, which links a person’s genetic makeup with their response to drugs. By analyzing genetic variants, AI models can predict how well a patient will respond to a specific medication, allowing doctors to personalize treatment regimens for maximum efficacy and minimal side effects.
- Predicting Disease Progression: AI can also analyze vast amounts of health data to predict how diseases will progress in different patients, enabling early intervention with targeted therapies. For example, AI models can track the progression of diseases like Alzheimer’s, cancer, and diabetes, helping physicians choose the best treatment strategies at each stage of the illness.
- Clinical Trial Matching: AI is increasingly being used to match patients with the most suitable clinical trials based on their unique genetic and medical profiles. This helps ensure that patients receive the most promising treatments and accelerates the development of drugs that may work for specific populations.
As AI continues to analyze patient data and refine treatment strategies, it holds the potential to provide a level of precision in healthcare that was previously unattainable.
Challenges and Limitations: Overcoming Data Biases and the Need for Human Oversight
While AI has great promise in revolutionizing drug discovery, it is not without its challenges and limitations:
- Data Bias: One of the major concerns in AI-driven drug discovery is the potential for bias in the data. If the data used to train AI models is incomplete or unrepresentative, the resulting predictions may not be accurate for all populations. For example, clinical trial data may be disproportionately based on certain ethnic groups, leading to drugs that are less effective or riskier for others. Addressing data diversity and ensuring fairness in AI models is critical to achieving equitable healthcare outcomes.
- Data Privacy and Security: The use of patient data in AI-driven drug discovery raises concerns about data privacy and security. Protecting sensitive health information while using it to train AI models is a challenge that requires careful regulation and robust cybersecurity measures.
- Human Oversight: While AI can enhance the drug discovery process, it is not a replacement for human expertise. Human oversight is essential to validate AI predictions, interpret complex medical data, and ensure that ethical considerations are upheld. For instance, regulatory bodies such as the FDA must assess the safety and efficacy of AI-powered drug candidates before they can be approved for clinical use.
- Interpretability: Many AI models, particularly deep learning models, are often seen as “black boxes,” meaning it is difficult to understand how they arrive at certain conclusions. This lack of transparency can make it challenging for researchers and healthcare professionals to trust AI recommendations fully, especially when it comes to life-or-death decisions.
Conclusion: The Future of AI in Revolutionizing Pharmaceuticals and Healthcare
AI is already making significant strides in transforming drug discovery, offering faster, more accurate predictions, and creating opportunities for personalized medicine. By unlocking new insights from vast datasets, AI is enabling the development of drugs that are more effective, with fewer side effects, and at a fraction of the cost and time of traditional methods. The combination of AI’s ability to analyze data, coupled with the expertise of researchers and clinicians, is propelling the pharmaceutical industry toward a new era of innovation.
Despite the challenges, AI’s potential to change healthcare is immense. From early disease detection to personalized treatments, AI could ultimately improve patient outcomes, increase access to life-saving drugs, and reduce the financial burden of drug development. As AI continues to evolve, it will play an increasingly central role in shaping the future of pharmaceuticals and healthcare.
Discussion about this post