Introduction: The Promise of Quantum Computing in the Era of AI
Artificial intelligence (AI) has made incredible strides in recent years, powering everything from autonomous vehicles and personalized recommendations to medical diagnostics and natural language processing. However, despite these advancements, the training of AI models remains a significant bottleneck. The process of training large-scale machine learning models requires immense computational resources, vast amounts of data, and extensive time to process. This is where quantum computing enters the conversation as a potential game-changer.
Quantum computing, which leverages the principles of quantum mechanics to process information, promises to revolutionize fields that rely on intense computational power, including AI. By performing certain calculations exponentially faster than classical computers, quantum computing could dramatically enhance the training efficiency of AI models. In this article, we will explore the breakthroughs in quantum computing, how they might impact AI model training, and whether this marks the dawn of a new era for machine learning.
Understanding Quantum Computing and Its Potential
At the heart of quantum computing is the concept of quantum bits, or qubits, which are the fundamental units of quantum information. Unlike classical bits, which can be in one of two states (0 or 1), qubits can exist in a state of superposition, meaning they can represent both 0 and 1 simultaneously. Additionally, through a phenomenon called entanglement, qubits can be interconnected, allowing them to perform complex computations in parallel that classical computers would struggle to match.
Quantum computers exploit these properties to potentially solve problems that would take classical computers millennia to address. For example, quantum computers could outperform traditional computers in areas such as factorization, optimization, and simulation of quantum systems.
But how does this relate to artificial intelligence?
The Challenges of Training AI Models
Training AI models, especially deep learning models, requires significant computational resources. Models like deep neural networks, transformers, and other large architectures have millions (or even billions) of parameters that need to be optimized through a process called gradient descent. This involves repeatedly adjusting the parameters based on data input, which can take an immense amount of time and computational power, especially when dealing with massive datasets.
The two major challenges in AI training today are:
1. Data-Intensive Nature of AI Models
AI models often require vast amounts of data to be trained effectively. For example, training a large language model like GPT-3 or a deep learning model for image recognition demands access to massive datasets of text, images, or video. Storing, managing, and processing these datasets requires huge computational resources.
2. Computational Cost of Model Training
The computational cost associated with training large AI models is significant. For instance, training state-of-the-art models can take weeks or even months on traditional graphics processing units (GPUs) or tensor processing units (TPUs), running continuously. The energy consumption and cost associated with this process are also growing concerns, especially for large tech companies and research institutions.
This is where quantum computing has the potential to step in and address these limitations.
Quantum Computing and AI: A Symbiotic Relationship
While quantum computing is still in its infancy, research has already demonstrated its potential to transform the efficiency of AI training in several key ways:
1. Faster Optimization Algorithms
One of the most promising applications of quantum computing in AI is its ability to accelerate the optimization process. Optimization is at the heart of AI model training: the goal is to find the best parameters that minimize error or loss in the model’s predictions. Classical optimization algorithms, such as gradient descent, are limited by the computational power available. In contrast, quantum computing could significantly speed up this process by using quantum algorithms such as Quantum Approximate Optimization Algorithm (QAOA) and Quantum Gradient Descent.
These quantum algorithms can explore the vast search space of possible solutions in parallel, potentially identifying optimal solutions more efficiently. This could reduce the time needed to train AI models and make it feasible to train even larger, more complex models.
2. Quantum Machine Learning Algorithms
Quantum computing has also given rise to a new branch of research known as Quantum Machine Learning (QML). QML seeks to integrate quantum computing with machine learning algorithms, offering the possibility of creating more efficient models for tasks such as classification, clustering, and regression.
For example, quantum computers can be used to construct quantum-enhanced neural networks, where quantum algorithms accelerate training by improving the way the model processes information. These quantum-enhanced networks could outperform traditional models on specific tasks, enabling them to learn faster and with less data.
3. Quantum Data Processing
Classical machine learning models require large datasets for training, and these datasets must be processed in classical ways. However, quantum computing offers the potential for quantum-enhanced data processing. With quantum algorithms like the Quantum Fourier Transform and Quantum Principal Component Analysis (PCA), quantum computers can process and analyze data in ways that classical computers cannot, potentially identifying patterns in data much more efficiently.
For example, quantum computers could be used to analyze complex datasets, such as those involving multiple variables or high-dimensional data, much faster than classical systems. This could allow AI models to train more effectively and with fewer computational resources.
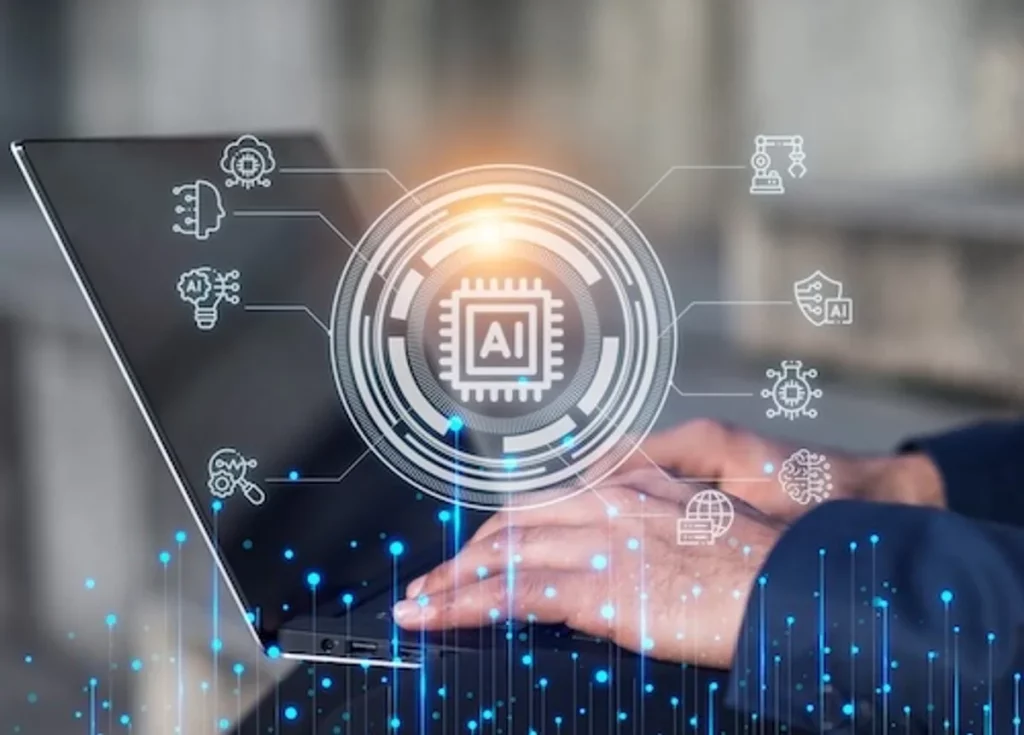
Practical Applications of Quantum Computing in AI Training
While the full integration of quantum computing into AI model training is still a long way off, researchers are already exploring some practical applications of quantum computing in AI. Some of the key areas where quantum computing could revolutionize AI model training include:
1. Accelerating Drug Discovery
Quantum computing has the potential to speed up the training of AI models in fields like pharmaceutical research and drug discovery. Traditional methods for simulating molecular interactions are time-consuming and computationally expensive. By using quantum computers to simulate quantum systems at a molecular level, researchers can create AI models that predict the interactions between different molecules, potentially discovering new drugs in a fraction of the time it would take classical computers.
2. Natural Language Processing (NLP)
Quantum computing could dramatically improve the efficiency of training large NLP models like BERT and GPT-3. NLP tasks such as machine translation, sentiment analysis, and language generation are highly data-intensive and computationally expensive. By speeding up the optimization process and enhancing data analysis capabilities, quantum computing could allow for faster development and more efficient use of NLP models.
3. Autonomous Systems and Robotics
AI plays a central role in autonomous systems and robotics, where efficient model training is essential. Quantum computing could be used to enhance the speed and accuracy of models that help robots understand their environment, make decisions, and execute tasks autonomously. By enabling faster model training, quantum computing could accelerate the development of autonomous vehicles, industrial robots, and even AI-driven drones.
Challenges and Limitations of Quantum Computing in AI Training
Despite its potential, quantum computing faces several challenges that must be addressed before it can revolutionize AI training:
1. Quantum Hardware Limitations
The current state of quantum hardware is still far from ideal. Most quantum computers today are still in the Noisy Intermediate-Scale Quantum (NISQ) era, meaning they are not yet capable of handling complex, real-world AI training tasks. Issues like quantum decoherence, error rates, and scalability must be overcome before quantum computers can reliably perform tasks at scale.
2. Algorithm Development
Developing quantum algorithms that can effectively speed up AI model training is still a work in progress. While quantum machine learning is a promising field, many quantum algorithms are still in the experimental stages, and there are significant challenges to making these algorithms robust enough for large-scale, real-world applications.
3. Integration with Classical Systems
For quantum computing to be practical in AI model training, it will need to integrate seamlessly with classical computing systems. Hybrid models, where quantum processors handle specific parts of the AI model training while classical systems manage the rest, may be the most realistic approach in the near future. However, this integration poses its own set of technical challenges.
Conclusion: A Quantum Leap for AI Training?
The breakthroughs in quantum computing are still emerging, but the potential to revolutionize AI model training is clear. Quantum computers promise to enhance optimization algorithms, improve machine learning processes, and enable faster data processing, ultimately leading to more efficient AI models. However, the practical application of quantum computing in AI training is still in its infancy, and several hurdles remain before quantum computers can fully integrate into mainstream AI development.
As research in both quantum computing and AI continues to evolve, it is likely that we will see more targeted, hybrid approaches that combine the best of both worlds—quantum computing and classical systems. If and when quantum computing becomes a powerful tool for AI training, we can expect faster, more efficient, and more scalable AI models, which could open up new frontiers in machine learning and transform industries ranging from healthcare to finance to autonomous technology.
The future of AI may very well be quantum-powered—ushering in a new era of machine learning and computational capabilities.
Discussion about this post