Introduction: The Use of AI in Transforming Drug Discovery
The field of drug discovery is undergoing a profound transformation, thanks to the power of Artificial Intelligence (AI). Traditional drug development processes, which can take decades and cost billions of dollars, are being accelerated by AI technologies, offering a faster, more efficient route to discovering new treatments. In recent years, machine learning models, deep learning algorithms, and advanced data analytics have become essential tools in pharmaceutical research, helping scientists and researchers identify promising drug candidates with unprecedented speed and accuracy.
AI’s ability to analyze vast amounts of biological and chemical data has revolutionized the way researchers approach drug discovery. From screening chemical compounds to predicting drug efficacy and patient response, AI is changing how pharmaceutical companies design, test, and bring new therapies to market. The result is not just faster drug development but also the potential for more personalized, precise treatments that could improve patient outcomes across various diseases, including cancer, neurological disorders, and chronic conditions.
This article explores the transformative impact of AI in drug discovery, including how AI models work, real-world case studies of AI-driven drug discoveries, the role of AI in personalized medicine, and the challenges the industry faces as it integrates AI technologies into the drug development pipeline.
How AI Models Work: Machine Learning and Data Analysis for Predicting Drug Efficacy
At the heart of AI-driven drug discovery are machine learning models and data analysis techniques that help researchers process and interpret vast datasets from a variety of sources, including genomics, clinical trials, and chemical databases. These AI models use statistical techniques to identify patterns, correlations, and predictive factors that would otherwise be difficult for human researchers to detect.
- Data Collection and Preprocessing: AI in drug discovery begins with gathering large-scale datasets from multiple sources, such as genetic data, clinical trial results, biochemical interactions, and chemical structures. The data is cleaned and preprocessed to ensure consistency and quality. This massive amount of information serves as the foundation for training AI algorithms.
- Training AI Models: Machine learning models are trained using historical data from previous drug development projects. By exposing the AI system to known outcomes—such as which compounds successfully treated certain diseases or which genetic mutations responded well to specific drugs—the system learns to recognize the variables that influence drug efficacy. The models use this data to identify new compounds that may have similar beneficial properties, improving the chances of success for future treatments.
- Drug Discovery and Screening: Once trained, AI models can screen millions of chemical compounds in virtual environments to predict which ones are most likely to be effective for treating specific diseases. These models simulate how molecules interact with proteins and other biological targets, providing valuable insights into which drugs might work in the real world. AI-driven drug discovery platforms, like those developed by companies such as Atomwise and Insilico Medicine, are able to predict the biological activity of compounds before they undergo costly and time-consuming clinical testing.
- Predicting Drug Efficacy: One of the most valuable aspects of AI in drug discovery is its ability to predict the efficacy of drug candidates in treating specific diseases. AI models can analyze complex biological data and identify patterns related to how diseases progress and how various molecules may interact with the body. By predicting how a drug will behave in a patient’s system, AI can help to identify the most promising candidates for further development, reducing the number of ineffective drugs that move forward in clinical trials.
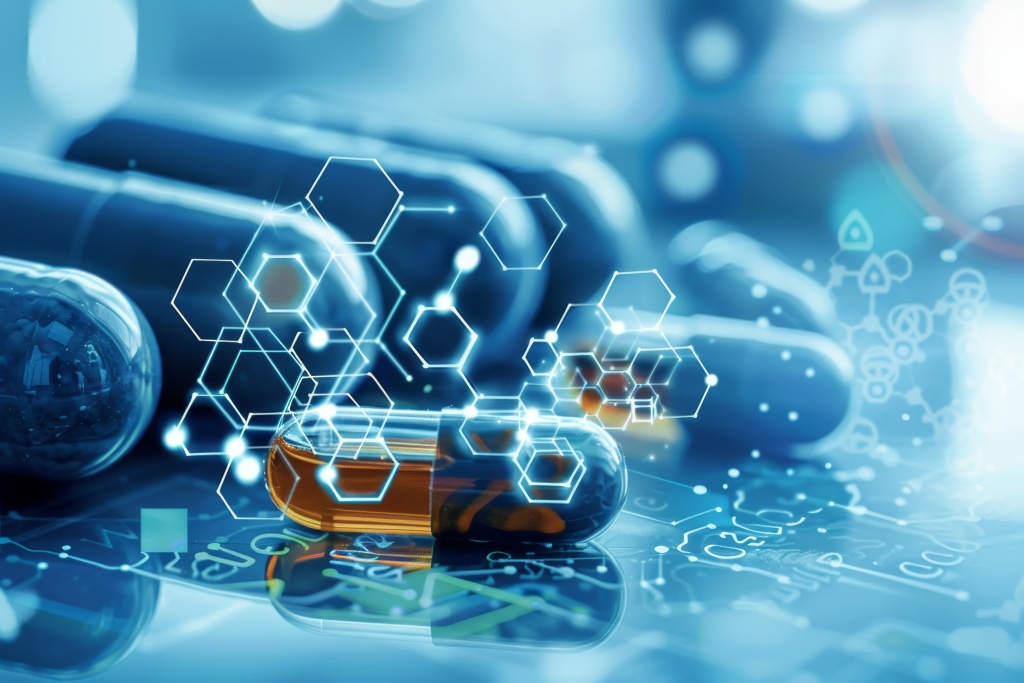
Case Studies: Successful AI-Driven Drug Discoveries in Recent Years
While the integration of AI in drug discovery is still relatively new, several high-profile successes demonstrate the potential of AI to speed up the process and improve outcomes. Some of the most notable case studies of AI-driven drug discoveries include:
- Insilico Medicine’s AI for Aging: Insilico Medicine, a biotechnology company, used AI to identify a potential drug for aging-related diseases. In 2019, the company successfully identified a novel drug candidate that could slow down the aging process by targeting specific cellular pathways. This discovery was made using the company’s deep learning algorithms, which analyzed a vast array of genomic and chemical data to pinpoint the best compounds for targeting the aging process.
- Atomwise and Ebola: Atomwise, a leader in AI-driven drug discovery, used its AI-powered platform to screen millions of compounds for potential treatments for Ebola. The company’s platform identified two existing drugs that could potentially treat the virus, cutting down the research time from months to just a few days. Atomwise’s success has shown how AI can rapidly find repurposed drugs that are already approved for other uses, speeding up the path to treatment during urgent health crises.
- BenevolentAI and COVID-19: In response to the global COVID-19 pandemic, BenevolentAI, a biotech company, used AI to identify promising drug candidates for treating COVID-19. The company’s algorithms analyzed scientific literature, clinical trial data, and genomic information to predict which existing drugs could potentially be repurposed to treat the virus. The company identified Baricitinib, a drug used for rheumatoid arthritis, as a promising candidate for treating COVID-19 patients, which was later authorized for emergency use by regulatory agencies.
These case studies illustrate how AI can accelerate the drug discovery process by rapidly identifying viable drug candidates, simulating interactions, and screening compounds with high efficiency.
AI in Personalized Medicine: Tailoring Treatments Using AI Insights from Patient Data
One of the most exciting prospects of AI in drug discovery is its ability to contribute to the growing field of personalized medicine. Personalized medicine involves tailoring treatments based on an individual’s genetic makeup, lifestyle, and specific disease characteristics, rather than using a one-size-fits-all approach. AI can play a pivotal role in enabling personalized treatment by analyzing patient data and identifying the most effective therapies for each individual.
- Genomic Data Analysis: AI models can analyze genomic data from patients to identify specific genetic mutations or biomarkers associated with diseases like cancer, diabetes, and cardiovascular conditions. This information can then be used to predict which drugs will be most effective for a patient based on their genetic profile. AI can also help in identifying new biomarkers, allowing for the development of more precise and targeted therapies.
- Predicting Treatment Response: AI can be used to analyze how different patients respond to specific treatments. By leveraging data from clinical trials, electronic health records, and genetic databases, AI can identify patterns in treatment efficacy and predict which patients are most likely to benefit from particular drugs. This allows clinicians to make more informed decisions, improving patient outcomes and reducing the risks of ineffective treatments.
- Optimizing Clinical Trials: Personalized medicine powered by AI can also enhance the design of clinical trials. AI can help identify suitable patient populations for trials, predict the most likely treatment outcomes, and even monitor patients in real-time to adjust the treatment protocol as needed. This can lead to more successful trials and faster approval of new drugs.
Challenges and Limitations: Overcoming Data Biases and the Need for Human Oversight
Despite its potential, AI-powered drug discovery is not without its challenges. Several obstacles must be overcome before AI can fully realize its transformative potential in pharmaceuticals.
- Data Biases: AI models are only as good as the data they are trained on. If the data used to train AI systems is biased or unrepresentative, the resulting predictions may not be accurate or applicable to diverse populations. For example, if clinical trial data predominantly comes from one demographic group, AI models may be less effective in predicting outcomes for underrepresented populations, such as ethnic minorities or people with certain pre-existing conditions.
- Interpretability: Many AI models, particularly deep learning algorithms, operate as “black boxes,” meaning it can be difficult to understand exactly how they arrive at specific predictions. This lack of transparency can be a significant barrier in the pharmaceutical industry, where the safety and efficacy of drugs are of paramount importance. Researchers and clinicians need to trust AI predictions, which requires greater interpretability and explainability from AI systems.
- Regulatory Hurdles: As AI continues to play a larger role in drug discovery, regulatory agencies must adapt to ensure that AI-driven drugs are safe and effective. This includes developing new standards for AI-based drug development, as well as providing clear guidelines for clinical trials and approval processes. Ensuring that AI-driven discoveries meet safety and efficacy standards will require collaboration between AI developers, pharmaceutical companies, and regulatory bodies.
- Human Oversight: While AI is a powerful tool, it is not infallible. Human oversight remains crucial to ensure that AI-driven discoveries are safe and ethically sound. Experts in biology, medicine, and pharmacology must work alongside AI systems to interpret results, validate predictions, and make informed decisions.
Conclusion: The Future of AI in Revolutionizing Pharmaceuticals and Healthcare
AI has the potential to completely transform the drug discovery process, speeding up the development of new treatments, enhancing personalized medicine, and improving patient outcomes. As machine learning algorithms continue to evolve, AI will become an even more integral part of the pharmaceutical industry, enabling faster, more efficient, and more targeted therapies.
However, challenges such as data biases, regulatory hurdles, and the need for human oversight must be addressed before AI can fully revolutionize drug development. The future of AI in pharmaceuticals looks bright, with vast potential to accelerate the search for new treatments and improve healthcare on a global scale. As AI technologies continue to advance, the intersection of machine learning, medicine, and patient care will usher in a new era of healthcare innovation.
Discussion about this post