Introduction: AI’s Pivotal Role in the Development of Self-Driving Cars
The dream of fully autonomous vehicles has been a long-standing goal in the automotive industry, but it is artificial intelligence (AI) that is finally turning this dream into a reality. AI technology has made remarkable strides in recent years, enabling vehicles to navigate roads, interpret data, and make decisions without human intervention. Autonomous vehicles are now equipped with AI-driven systems that allow them to recognize road signs, detect pedestrians, navigate complex traffic situations, and make real-time decisions—all while adhering to traffic laws and ensuring passenger safety.
The promise of autonomous vehicles (AVs) extends beyond convenience. By eliminating human error, which is responsible for the vast majority of traffic accidents, AI has the potential to make transportation safer, more efficient, and more sustainable. This article explores how AI is playing a central role in the development of self-driving cars, the safety features it enables, the challenges to achieving full autonomy, and how AI can reshape the future of transportation.
AI and Sensor Fusion: How Autonomous Vehicles Use AI to Process Information from Various Sensors
At the heart of autonomous vehicle technology is the integration of multiple sensors that allow the car to “see” and interpret the environment around it. These sensors include cameras, radar, LiDAR (Light Detection and Ranging), ultrasonic sensors, and GPS. However, it is not just the sensors themselves that make a vehicle autonomous—it’s the AI algorithms that process and integrate data from all these sensors to form a cohesive understanding of the vehicle’s surroundings. This process, known as sensor fusion, is a key aspect of self-driving technology.
- Data Collection and Processing: Autonomous vehicles are equipped with a range of sensors that continuously gather data from their surroundings. Cameras provide high-definition images, radar detects objects at a distance, LiDAR creates detailed 3D maps of the environment, and ultrasonic sensors measure proximity to nearby objects. GPS provides location data for navigation, while AI algorithms process this data to create a real-time map of the vehicle’s surroundings.
- Sensor Fusion: AI uses sensor fusion to combine the data from all of these sensors, taking the strengths of each technology and compensating for their weaknesses. For example, while LiDAR offers precise 3D depth information, it may struggle in low-visibility conditions like heavy rain or fog. Radar, on the other hand, can perform well in such conditions but lacks the precision of LiDAR. By combining these sources of data, AI ensures a more accurate and reliable understanding of the vehicle’s environment.
- Real-Time Decision Making: Once the data has been processed and fused, AI algorithms analyze the information to make decisions about how the vehicle should behave. For example, AI can identify pedestrians on the road, predict the behavior of other vehicles, detect obstacles, and interpret traffic signals. These real-time decisions ensure that the vehicle can react quickly to changing conditions, enabling safe navigation in complex environments.
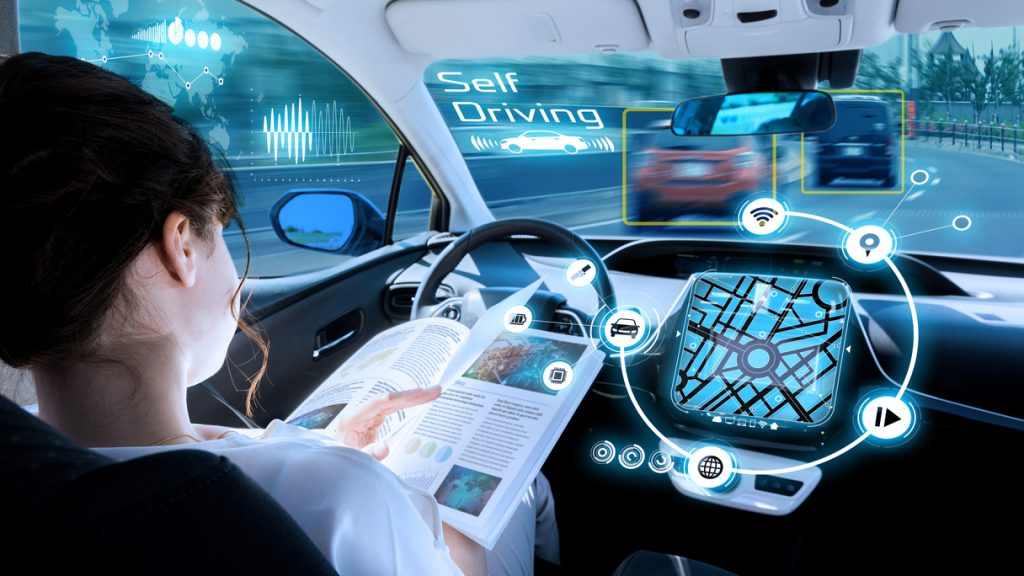
Safety Features and Performance: AI Algorithms Ensuring Safer Driving and Accident Prevention
One of the primary motivations for developing autonomous vehicles is the potential to significantly reduce traffic accidents, many of which are caused by human error. AI-powered systems are designed to prevent accidents by detecting hazards and reacting faster than human drivers ever could.
- Collision Avoidance: AI algorithms are capable of processing massive amounts of data from the vehicle’s sensors in real time, identifying potential hazards like pedestrians, other vehicles, cyclists, or road debris. For example, if an AI system detects a car unexpectedly swerving into the vehicle’s path, it can automatically apply the brakes or steer the car to avoid the collision, even before a human driver could react.
- Adaptive Cruise Control and Lane Keeping: Many autonomous vehicles are already equipped with AI-powered safety features like adaptive cruise control and lane-keeping assist. These systems allow the car to maintain a safe distance from the vehicle in front, adjust its speed based on traffic conditions, and keep the vehicle centered within its lane. These systems can reduce the risk of accidents caused by tailgating, drifting out of lane, or failing to adjust speed to match traffic flow.
- Predictive Analytics: AI also uses predictive analytics to foresee potential risks before they become immediate threats. By analyzing patterns in driving behavior, traffic conditions, and environmental factors, AI can make anticipatory decisions that prevent accidents. For example, if a vehicle is approaching an intersection where another car is speeding through a red light, AI might slow the car down to avoid a possible collision.
- Emergency Response Systems: In the event of a medical emergency or other unforeseen circumstances, autonomous vehicles can rely on AI-powered emergency response systems that can safely guide the vehicle to a stop. These systems are designed to prioritize the safety of both the passengers and pedestrians, ensuring that the vehicle does not cause further harm in the event of an emergency.
Challenges to Full Autonomy: Legal, Ethical, and Technological Barriers
While AI has made significant advancements in autonomous driving, achieving full autonomy—where the vehicle can operate without any human intervention—is still fraught with challenges. These obstacles are not only technological but also legal, ethical, and regulatory in nature.
- Technological Limitations: Despite the advancements in AI and sensor technology, autonomous vehicles still face challenges in certain situations. For example, self-driving cars may struggle in adverse weather conditions, such as heavy snow, rain, or fog, where sensors may not work as effectively. Complex urban environments with unpredictable traffic, cyclists, and pedestrians also pose challenges. AI systems must be capable of reacting to a wide variety of real-world conditions, which requires constant innovation and refinement of algorithms.
- Legal and Regulatory Issues: Governments around the world are working to establish regulations for autonomous vehicles, but there is no universal framework. Questions about liability in the event of an accident, insurance policies, and the testing and deployment of self-driving cars remain unresolved. Additionally, ethical dilemmas—such as how an autonomous vehicle should behave in situations where an accident is unavoidable—are prompting widespread debate among lawmakers, ethicists, and engineers.
- Ethical Concerns: The ethical questions surrounding autonomous vehicles are complex. For example, in an unavoidable crash, how should an AI system decide whom to harm? Should the car prioritize the safety of its passengers over pedestrians? These types of “trolley problem” scenarios are a significant ethical hurdle for autonomous vehicle developers. AI will need to be programmed with clear guidelines to ensure these decisions are made responsibly, though the answer may vary depending on cultural and legal norms.
AI and Traffic Management: How AI Can Optimize Traffic Flow for Autonomous Fleets
In addition to improving the safety and performance of individual vehicles, AI has the potential to optimize traffic flow and reduce congestion across entire cities. The proliferation of autonomous vehicles could lead to the development of autonomous fleets—vehicles that communicate with each other and with traffic management systems to ensure smooth, efficient travel.
- Vehicle-to-Vehicle (V2V) Communication: One of the major advantages of autonomous vehicles is their ability to communicate with each other in real time. AI can enable vehicle-to-vehicle (V2V) communication, allowing cars to share information about road conditions, traffic, accidents, or upcoming hazards. This collaboration could lead to smoother, more synchronized traffic flow, reducing bottlenecks and preventing accidents caused by human misjudgment.
- AI in Traffic Management Systems: Autonomous vehicles could also interact with city-wide traffic management systems, which are powered by AI algorithms. These systems could adjust traffic signals, monitor congestion, and optimize routes for autonomous fleets. For example, if a major intersection is congested, AI could reroute autonomous vehicles to less crowded streets, minimizing travel time and reducing fuel consumption.
- Reducing Traffic Accidents and Emissions: By coordinating traffic flow and improving the efficiency of roads, AI can also reduce the environmental impact of transportation. Fewer traffic jams and smoother commutes mean fewer emissions, contributing to a greener, more sustainable transportation ecosystem.
Conclusion: The Path Forward for AI in Autonomous Vehicles
The future of transportation is on the cusp of a major transformation, driven largely by artificial intelligence. Self-driving cars powered by AI have the potential to reduce accidents, alleviate traffic congestion, and create a more sustainable, efficient transportation system. However, full autonomy requires overcoming technological limitations, addressing legal and ethical concerns, and ensuring that autonomous vehicles are integrated into the broader infrastructure of cities and highways.
As AI continues to evolve, autonomous vehicles will likely become an integral part of everyday life. Their ability to analyze real-time data, make split-second decisions, and communicate with other vehicles will fundamentally change how we think about mobility. While there are challenges ahead, the path toward a safer, smarter future of autonomous driving is becoming clearer—and AI will be the driving force behind it.
Discussion about this post