In recent years, the healthcare industry has seen transformative changes driven by artificial intelligence (AI). Among the most promising advancements is AI’s ability to revolutionize health diagnostics, enabling faster, more accurate, and more efficient disease detection. By leveraging machine learning algorithms and vast datasets, AI is helping clinicians identify conditions earlier, leading to better patient outcomes and reduced healthcare costs. This article explores how AI is reshaping the landscape of health diagnostics and enhancing early detection capabilities across a variety of medical conditions.
1. The Role of AI in Health Diagnostics
AI refers to the simulation of human intelligence in machines that are programmed to think and learn like humans. When applied to healthcare diagnostics, AI enables the analysis of medical data—such as medical imaging, genetic information, and patient history—with greater speed and accuracy than traditional methods. AI can identify patterns and anomalies within this data that might be difficult for the human eye to spot, providing clinicians with insights that enhance their ability to diagnose conditions early.
Machine Learning and Deep Learning:
At the core of AI-powered diagnostics are machine learning (ML) and deep learning (DL) algorithms. Machine learning allows systems to learn from historical data and improve their predictions over time. Deep learning, a subset of machine learning, uses multi-layered neural networks to analyze complex data such as medical images or genomic data, mimicking how the human brain processes information.
These algorithms continuously improve as they process more data, allowing them to identify emerging trends, predict future health risks, and flag abnormal findings that may indicate the onset of disease. As a result, AI tools can assist healthcare professionals in providing early interventions, which can be critical in improving patient outcomes.
2. Faster, More Accurate Disease Detection
AI has shown great potential in improving the speed and accuracy of disease detection across several medical fields, ranging from radiology and oncology to cardiology and neurology. Below are some key examples of how AI is enhancing disease detection:
Radiology and Imaging:
One of the most significant applications of AI in healthcare is in the field of medical imaging. Radiologists often rely on X-rays, CT scans, MRIs, and other imaging techniques to detect conditions like cancer, fractures, and cardiovascular diseases. However, these images can be complex and challenging to analyze, especially when dealing with large volumes of data.
AI algorithms can rapidly process medical images and identify subtle abnormalities with exceptional accuracy. For example, AI systems trained on thousands of medical images can identify early signs of conditions such as breast cancer, lung cancer, or brain tumors that might be missed by human radiologists. AI tools are now capable of detecting these conditions at earlier stages, improving the chances of successful treatment.
Additionally, AI-powered diagnostic tools can help radiologists prioritize cases, flagging the most urgent images that require immediate attention, and ensuring that resources are allocated effectively.
Oncology:
In oncology, early cancer detection is crucial for improving survival rates. AI is playing a vital role in detecting cancerous tumors and precancerous lesions in a range of organs, including the lungs, breast, and skin.
For instance, AI algorithms have been developed to analyze mammograms and detect signs of breast cancer at earlier stages than traditional methods. AI tools can identify minute changes in tissue patterns, leading to earlier detection and a better prognosis for patients. Similarly, AI is being used to detect skin cancer by analyzing images of moles and lesions, helping dermatologists identify melanoma before it spreads.
AI is also being employed in the analysis of biopsy samples and genomic data to identify genetic mutations and predict the likelihood of developing cancer, allowing for personalized treatment plans based on the patient’s unique genetic makeup.
Cardiology:
AI is making significant strides in cardiovascular diagnostics by improving the detection of heart conditions such as heart disease, arrhythmias, and stroke risk. By analyzing data from electrocardiograms (ECGs), echocardiograms, and other cardiovascular tests, AI can predict the risk of heart attacks and other cardiovascular events.
AI algorithms are particularly effective in detecting atrial fibrillation (AF), an irregular heartbeat that can lead to strokes. Machine learning models trained on ECG data can identify patterns associated with AF, enabling early intervention and reducing the risk of complications.
In addition, AI is being integrated into wearable devices, such as smartwatches, that monitor heart rate and detect early signs of heart-related issues. These devices can alert users to irregularities in their heart rhythm, prompting them to seek medical attention before a more serious event occurs.
Neurology:
In the field of neurology, AI is helping to detect neurological diseases such as Alzheimer’s disease, Parkinson’s disease, and multiple sclerosis at earlier stages. By analyzing brain scans, cognitive test scores, and genetic data, AI can identify patterns that suggest the onset of neurological conditions before symptoms become noticeable.
For example, AI models trained on brain MRI scans can detect early signs of Alzheimer’s disease, such as hippocampal shrinkage, which may be imperceptible to human observers. Early detection allows for earlier intervention, potentially slowing the progression of the disease and improving quality of life.
In Parkinson’s disease, AI algorithms are being used to analyze movement patterns through wearable sensors or video analysis, helping doctors diagnose the condition with greater accuracy. This can lead to early treatment and improved outcomes for patients.
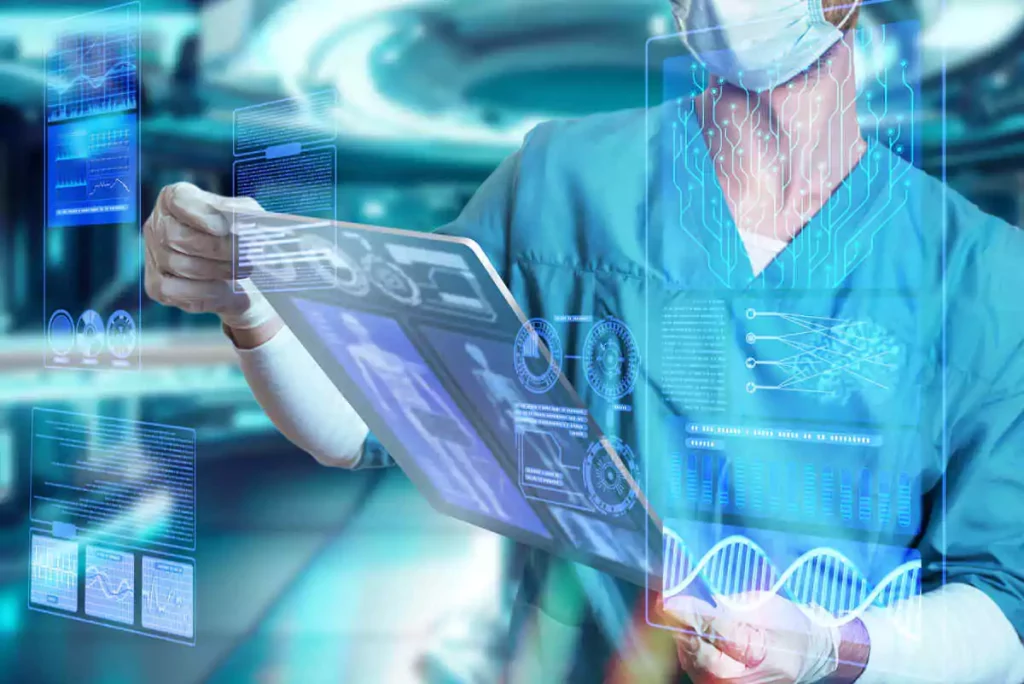
3. AI in Personalized Medicine and Risk Prediction
Beyond diagnostic applications, AI is also playing a critical role in personalized medicine. By analyzing an individual’s medical history, genetic information, and lifestyle factors, AI algorithms can provide insights into a person’s risk profile for developing specific diseases. This allows for preventative measures to be taken based on a person’s unique genetic makeup and health history.
Predictive Analytics:
AI-powered predictive analytics tools can assess the likelihood of future health events, such as heart attacks, strokes, or the development of chronic conditions like diabetes. These tools use a variety of data sources, including electronic health records (EHRs), medical imaging, and genomic data, to generate risk profiles for individual patients.
For example, in diabetes management, AI systems can analyze patterns in blood glucose levels, lifestyle factors, and other relevant data to predict future spikes or drops in blood sugar. This enables patients to take preventative measures before their condition worsens, reducing the risk of complications such as kidney failure or blindness.
Pharmacogenomics:
AI is also being used to enhance pharmacogenomics, the study of how genes affect an individual’s response to drugs. By analyzing genetic data, AI systems can identify which medications are most likely to be effective for a patient and which may cause adverse reactions. This information helps doctors make more informed decisions about prescribing drugs, ultimately improving treatment outcomes and reducing the risk of side effects.
4. Challenges and Ethical Considerations
While AI has tremendous potential to improve healthcare diagnostics, there are still several challenges to overcome. One of the biggest challenges is the quality and availability of data. AI algorithms rely on large datasets to learn and improve, but not all healthcare institutions have access to high-quality, diverse, and representative data. Inadequate data can lead to inaccurate predictions and diagnostic errors.
Data privacy is another concern. The use of personal health data for AI training raises questions about patient consent and data security. Ensuring that healthcare data is protected and used ethically is paramount to gaining the trust of patients and healthcare providers.
Additionally, bias in AI algorithms is a potential issue. If AI systems are trained on biased datasets, they may produce skewed results that disproportionately affect certain populations. Addressing these biases and ensuring that AI models are tested on diverse patient populations is crucial to preventing disparities in healthcare.
5. The Future of AI in Health Diagnostics
The future of AI in health diagnostics looks incredibly promising. As AI continues to advance, we can expect even more sophisticated algorithms capable of diagnosing a wider range of diseases with greater accuracy. In addition, the integration of AI with wearable health technology, genetic testing, and big data analytics will further enhance early detection capabilities and provide patients with personalized, real-time health insights.
In the coming years, AI-powered health diagnostics will likely become an essential part of routine medical care. With the potential to save lives through early detection, improve treatment outcomes, and reduce healthcare costs, AI is set to play a transformative role in the future of healthcare.
Discussion about this post