Introduction: Human Bias in Decision-Making and the Hope That AI Can Be Impartial
Human decision-making has always been influenced by a variety of biases. Whether implicit or explicit, these biases can result in decisions that are unfair, discriminatory, and irrational. These biases often manifest in critical areas such as hiring, lending, legal judgments, and healthcare, where the consequences of biased decisions can be profound and wide-reaching. In the modern world, AI is increasingly being viewed as a potential solution to combat human bias in decision-making processes. The hope is that AI, with its data-driven nature, can eliminate or at least reduce the influence of human bias, leading to fairer and more objective decisions.
However, while AI has the potential to be impartial and efficient, it is important to recognize that the systems that power AI are not immune to bias themselves. Bias can be embedded into AI systems through the data they are trained on, the design of the algorithms, and the decisions made by the developers who create them. As AI technologies continue to evolve, it is crucial to understand the complexities of human and AI bias and explore ways to mitigate its impact on decision-making processes.
This article will explore the relationship between human bias and AI, how AI can potentially reduce bias, the challenges of achieving impartiality in AI systems, and real-world case studies where AI is being used to address bias in decision-making.
Understanding Bias in AI: How Biases in Training Data Can Lead to Biased AI Systems
One of the central issues with AI and bias is the fact that AI systems are only as good as the data they are trained on. If the data used to train AI models is biased, then the AI system will inherit those biases. This is known as “data bias” and is one of the most common ways that AI can perpetuate or even exacerbate human biases. Bias in data can come from many sources: historical inequalities, societal prejudices, and the selection of non-representative data, among others. If these biases are not addressed, the AI model can make decisions that disproportionately favor one group over another.
A classic example of this issue has been seen in hiring algorithms, which are increasingly being used by companies to streamline the recruitment process. If an AI hiring system is trained on historical hiring data from a company that has traditionally favored male candidates over female candidates, the system may unintentionally perpetuate this bias by favoring male applicants. Similarly, if an AI system is trained on data that over-represents certain demographic groups (e.g., young, white candidates), it may develop preferences for those groups, leaving out qualified candidates from underrepresented communities.
Bias can also manifest in legal sentencing and judicial decisions. AI-based tools are used in some regions to assist judges in determining the appropriate sentence for a convicted individual. However, if the historical data used to train these tools contains biased patterns—such as a tendency to impose harsher sentences on certain racial or ethnic groups—the AI may replicate these biased decisions. A notable example is the case of COMPAS, a predictive tool used in the U.S. justice system to assess the risk of recidivism. Studies have shown that COMPAS tends to overestimate the risk of recidivism for Black defendants, leading to biased outcomes.
Another area where bias can occur is in facial recognition technology. AI-based facial recognition systems have been shown to exhibit bias based on race and gender. For example, studies have demonstrated that facial recognition software is less accurate at identifying the faces of people of color and women compared to white males. This has led to concerns over the fairness and reliability of facial recognition systems, particularly when used in law enforcement or security settings.
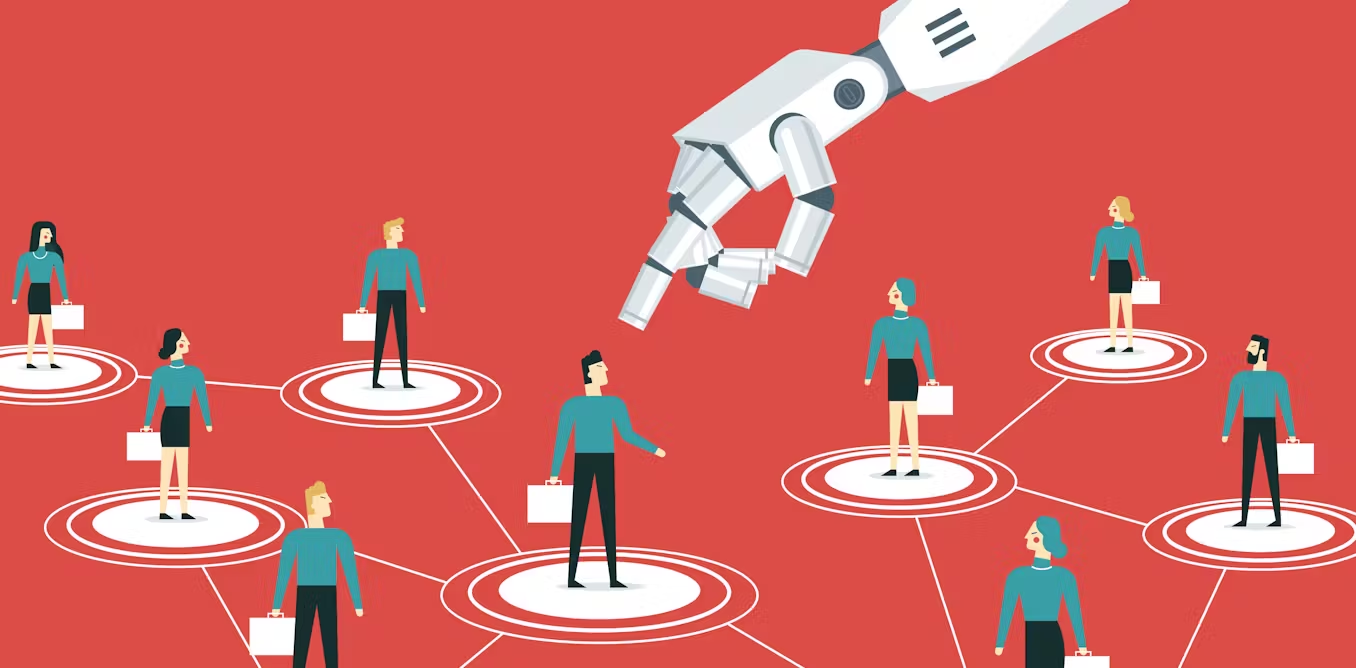
AI Solutions to Bias: Fairness Algorithms, Ensuring Diverse and Representative Data Sets, Transparency, and Ethical AI Frameworks
To mitigate bias in AI, researchers and developers are working on a variety of solutions that focus on improving the fairness, transparency, and accountability of AI systems. One of the most promising approaches is the development of fairness algorithms, which are designed to detect and reduce bias in AI models. These algorithms can help ensure that the outputs of an AI system are fair and equitable by identifying patterns of discrimination and adjusting for them in the decision-making process.
Another important solution is the use of diverse and representative data sets. The data used to train AI systems must accurately reflect the diversity of the population it will serve. If certain groups are underrepresented in the training data, the AI system may develop biased behaviors that disadvantage those groups. By ensuring that data sets are diverse and inclusive, developers can help reduce the risk of bias in AI models. This may involve actively seeking out underrepresented groups or data points and making sure that the AI system is trained on a broad range of information.
Transparency is also a crucial element in addressing bias in AI. Many AI systems, especially those based on deep learning, operate as “black boxes,” meaning that it is difficult to understand how they arrive at their decisions. This lack of transparency can be problematic, especially in high-stakes areas like hiring, healthcare, and criminal justice, where the consequences of biased decisions can be severe. To combat this, there is a growing focus on developing explainable AI (XAI) systems, which aim to provide insight into how AI models make their decisions. By making AI decisions more understandable, XAI can help ensure that these systems are not only fair but also accountable.
The development of ethical AI frameworks is another important step toward reducing bias in AI. Ethical AI frameworks are designed to guide the responsible development and deployment of AI technologies, ensuring that they are aligned with human values and societal norms. These frameworks often focus on principles such as fairness, accountability, transparency, and non-discrimination, and they provide developers with guidelines for creating AI systems that minimize the risk of bias. Organizations like the IEEE and the European Union have already begun to develop ethical guidelines for AI, and these efforts are likely to continue to grow in importance as AI becomes more pervasive in society.
Challenges: Overcoming Entrenched Biases in Data, Ensuring AI’s Decisions Align with Human Values, and Implementing Unbiased Algorithms at Scale
Despite the promising solutions outlined above, there are significant challenges to overcome in the quest to eliminate bias in AI. One of the biggest obstacles is overcoming entrenched biases in historical data. Biases that have existed in society for generations—such as gender and racial biases—are often reflected in the data used to train AI systems. These biases can be difficult to identify and address, especially if they are deeply ingrained in the data. Moreover, it may be challenging to determine how much historical bias should be corrected for, as doing so could unintentionally introduce new biases or inaccuracies into the system.
Another challenge is ensuring that AI’s decisions align with human values. AI systems are designed to make decisions based on the data they are trained on, but these decisions are not always aligned with human ethical principles. For example, an AI model might make a decision that is statistically optimal but morally questionable. For instance, an algorithm used in healthcare might prioritize cost-saving measures over patient well-being, resulting in poor health outcomes for certain groups. To address this, developers must ensure that AI systems are designed to prioritize human-centered values, such as fairness, equity, and well-being.
Implementing unbiased algorithms at scale is also a significant challenge. While fairness algorithms and diverse data sets can help reduce bias in AI systems, it can be difficult to implement these solutions across the wide variety of industries and applications that use AI. Ensuring that AI systems are consistently fair, transparent, and accountable requires ongoing monitoring, evaluation, and adaptation. Additionally, as AI systems are deployed at scale, there is a risk that small biases in the data or algorithmic design could have a magnified impact on society as a whole.
Case Studies: Real-World Examples of AI Efforts to Reduce Bias (e.g., IBM Watson for Healthcare, AI Ethics in Hiring Algorithms)
Several companies and organizations are making strides in addressing AI bias through real-world applications. One of the most well-known efforts is IBM Watson for Healthcare, which aims to assist medical professionals in making more accurate and unbiased treatment decisions. IBM has worked to ensure that Watson is trained on diverse data sets that reflect a wide range of patient demographics, ensuring that it can make recommendations that are inclusive and equitable. Watson’s AI-powered platform has been used to analyze medical records, suggest treatment plans, and even assist in cancer diagnosis, all while striving to minimize biases in the data and algorithmic decisions.
Another example is the use of AI in hiring algorithms. Many companies are developing AI-driven hiring tools that aim to reduce human bias in the recruitment process. These systems analyze resumes and applications to identify the most qualified candidates, potentially eliminating unconscious biases related to gender, race, or ethnicity. However, these systems also require careful monitoring to ensure that they do not replicate or exacerbate existing biases. Companies like Unilever have been experimenting with AI to assess candidates based on their skills and potential, rather than traditional resume-based hiring methods, helping to reduce bias in the recruitment process.
Conclusion: While AI Offers the Potential to Reduce Human Bias, Achieving Truly Unbiased AI Requires Continuous Improvement and Ethical Oversight
AI has the potential to significantly reduce human bias in decision-making, but achieving truly unbiased AI is a complex challenge. While fairness algorithms, diverse data sets, and ethical AI frameworks can help reduce bias, there are still many hurdles to overcome. Biases in historical data, the alignment of AI decisions with human values, and the scalability of unbiased algorithms remain key obstacles.
To ensure that AI systems are fair and impartial, continuous improvement, oversight, and accountability are required. As AI technologies continue to evolve and become more integrated into decision-making processes, it is essential that developers, regulators, and organizations work together to create a future where AI can truly be impartial, ethical, and beneficial for all.
Discussion about this post