Introduction: AI’s Role in Advancing Scientific Discovery and Innovation
In recent years, Artificial Intelligence (AI) has become one of the most transformative tools in scientific research, unlocking new potential for advancements across a variety of disciplines. From accelerating discoveries in medicine to optimizing climate models, AI has proven to be a game-changer in how scientists approach problem-solving, data analysis, and experimentation.
The role of AI in scientific research is multifaceted, offering both immediate and long-term benefits. AI is helping researchers process vast amounts of data, identify patterns and insights that were once impossible for humans to discern, and even predict future outcomes. This not only speeds up the scientific process but also enhances the precision of results, allowing for more effective innovations. As we continue to explore the integration of AI into the scientific realm, its impact grows more profound.
This article will examine the various benefits AI brings to scientific research, including how it revolutionizes data analysis, drug discovery, and medical research. We will also discuss the challenges associated with AI’s use in research, including biases, ethical concerns, and the need for transparency. The conclusion will reflect on how AI is poised to continue reshaping scientific discovery, provided its integration is approached with caution and responsibility.
AI in Data Analysis: The Ability to Analyze Massive Datasets, Uncovering Patterns and Insights Too Complex for Humans
One of the most significant benefits AI brings to scientific research is its ability to analyze large and complex datasets. Modern science is generating data at an unprecedented rate, and traditional methods of analysis are often too slow, too limited, or incapable of handling the sheer volume and complexity of the information. AI, particularly machine learning (ML) and deep learning, has the capacity to process and analyze this data quickly and efficiently, often uncovering insights that would otherwise be missed.
- Speed and Efficiency in Data Processing
AI’s ability to quickly process vast amounts of data is crucial for advancing research in fields such as genomics, climate science, physics, and social sciences. For example, in genomics, researchers are sequencing the human genome and studying genetic variations at a rate that would have been unimaginable a few decades ago. AI algorithms can quickly process the huge datasets generated by gene sequencing and identify patterns that may be associated with certain diseases or traits. This can significantly speed up the process of understanding genetic predispositions and potential therapies.
- Identifying Hidden Patterns
AI’s ability to detect patterns in large datasets is another key benefit. Traditional statistical methods often fall short when it comes to identifying complex relationships within data. AI, particularly machine learning algorithms, can sift through data in ways that humans cannot, identifying correlations, causal factors, and even predictive insights that might have gone unnoticed. For instance, in climate science, AI models are being used to predict weather patterns, track climate change, and model environmental factors with a level of precision that is unmatched by traditional approaches.
- Predictive Capabilities
Machine learning algorithms can also be used to make predictions based on historical data. In fields like epidemiology, AI models are used to predict the spread of diseases and identify potential hotspots for infection. Similarly, in materials science, AI can predict the properties of new materials based on historical data and simulations, helping researchers to develop new substances for applications in everything from renewable energy to medicine. The ability to make predictions based on existing data accelerates research and opens up new avenues for scientific discovery.
AI in Drug Discovery: Accelerating the Process of Finding New Treatments and Personalized Medicine
The pharmaceutical industry has traditionally faced lengthy and expensive processes in discovering and developing new drugs. AI has the potential to significantly speed up this process, leading to faster discoveries of effective treatments and therapies, with the added benefit of personalized medicine.
- Faster Drug Development
AI is already being used to optimize the process of drug discovery, reducing the time and cost involved. By analyzing biological data and predicting how different molecules might interact with human cells, AI can help identify promising drug candidates more quickly than traditional methods. In some cases, AI has already been used to discover new compounds for diseases that were previously difficult to treat, such as certain types of cancer and neurological disorders.
For example, researchers are using AI algorithms to identify new molecular structures that could lead to the development of novel antibiotics to combat antibiotic-resistant bacteria. In some cases, AI has helped researchers design molecules that are more effective at targeting specific types of cancer cells, significantly improving the chances of finding effective treatments in a fraction of the time it would take using traditional methods.
- Personalized Medicine
AI is also helping to pave the way for personalized medicine, where treatments are tailored to an individual’s specific genetic makeup. By analyzing the vast amounts of data available from genomics and patient records, AI can help doctors identify the most effective treatment plans for individual patients. This approach not only improves outcomes for patients but also helps reduce healthcare costs by ensuring that treatments are targeted and effective, rather than trial-and-error approaches that may lead to unnecessary side effects or ineffective treatments.
AI can also help identify patients who are at higher risk for certain conditions based on their genetic profiles, enabling earlier interventions and more proactive treatments. This leads to better health outcomes and a more efficient healthcare system overall.
- Drug Repurposing
Another exciting possibility in drug discovery involves AI’s ability to identify opportunities for drug repurposing. This means using existing drugs in new therapeutic areas, which can save both time and money in the drug development process. AI algorithms can analyze vast datasets to identify drugs that may already be approved for one condition but could potentially be effective for another. This has the potential to significantly accelerate the time to market for new treatments.
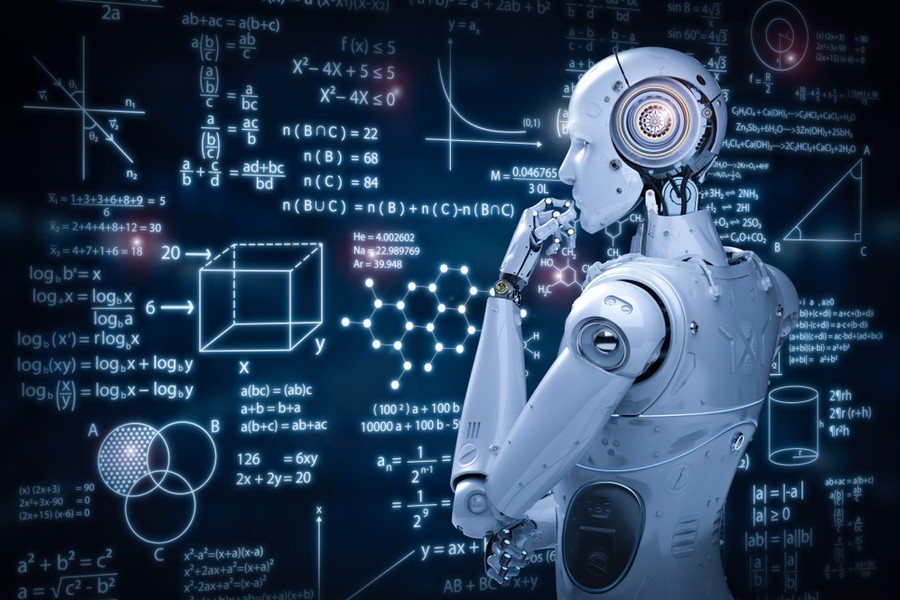
Challenges: Ensuring Transparency, Addressing Bias in Research, and the Need for Explainable AI
While AI’s benefits in scientific research are evident, there are several challenges that need to be addressed to ensure its responsible and effective integration. These challenges include ensuring transparency in AI models, addressing biases in research, and ensuring that AI systems are explainable and understandable.
- Transparency in AI Models
One of the main challenges with AI in scientific research is the “black-box” nature of many machine learning models. These models, particularly deep learning algorithms, can be difficult to interpret, making it challenging for scientists to fully understand how decisions are being made. This lack of transparency can be problematic in fields like healthcare, where understanding how an AI system arrived at a particular decision can be critical for patient safety.
For example, when AI is used to make medical diagnoses, it’s crucial that the model’s decision-making process is transparent so that doctors can trust the AI’s recommendations. As AI continues to play a larger role in healthcare, transparency and interpretability must be prioritized to ensure that AI systems can be trusted to make critical decisions.
- Addressing Bias in Research
Another significant concern is the potential for bias in AI models. Machine learning algorithms are only as good as the data they are trained on, and if the data is biased, the AI model can perpetuate those biases. This can lead to skewed results and potentially harmful conclusions, especially in sensitive areas like medical research.
For instance, if an AI model is trained on medical data that is predominantly from one demographic, such as Caucasian patients, the model may not perform as well for patients from other demographic groups. This can lead to inequities in healthcare outcomes, particularly if the model is used to inform treatment decisions.
- The Need for Explainable AI
Explainable AI (XAI) is an area of growing importance in scientific research. It refers to AI systems that are designed to provide clear explanations of their decision-making processes. This is particularly important in fields like healthcare, where decisions can have life-or-death consequences. Researchers and clinicians need to understand why an AI model has made a particular prediction or recommendation in order to trust and validate its output.
As AI continues to be used in high-stakes domains, ensuring that models are not only accurate but also explainable will be essential for fostering trust and accountability in scientific research.
Conclusion: AI Is Revolutionizing Scientific Research by Enhancing Speed and Precision, but Its Integration Must Be Carefully Managed to Prevent Bias and Ethical Concerns
AI has already begun to reshape the landscape of scientific research, providing unprecedented capabilities for data analysis, drug discovery, and personalized medicine. By helping researchers analyze massive datasets, uncover hidden patterns, and accelerate the discovery of new treatments, AI is accelerating the pace of scientific innovation.
However, the integration of AI into research must be approached carefully to mitigate potential risks, including transparency issues, biases in research, and ethical concerns about how AI decisions are made. While the technology is still evolving, AI’s potential to revolutionize scientific discovery is undeniable. With continued advancements, responsible implementation, and careful oversight, AI will continue to drive progress in a wide range of scientific fields, opening up new possibilities for innovation and improving the quality of life for people around the world.
Discussion about this post