Introduction: The Rise of AI in Healthcare
Artificial Intelligence (AI) has made substantial strides in recent years, with applications transforming industries from finance to entertainment. One area that has garnered significant attention is healthcare, where AI’s potential to enhance medical diagnosis, treatment, and patient care is increasingly recognized. AI systems, specifically those utilizing machine learning (ML) and deep learning (DL) algorithms, are being trained on vast datasets to detect diseases, predict health outcomes, and assist in decision-making.
From detecting cancer through medical imaging to analyzing genomic data for personalized medicine, AI systems are already proving their capability in medical diagnostics. However, a pressing question persists: Can AI truly replace human doctors’ expertise in making critical healthcare decisions, or will it always serve as a supplementary tool to enhance the decision-making process?
This article explores the accuracy of AI in medical diagnosis, the challenges involved, and whether AI can ever fully replace the judgment of human healthcare professionals.
The Role of AI in Medical Diagnosis
AI technologies in healthcare primarily work by analyzing large datasets, recognizing patterns, and making predictions or classifications based on that data. Some common AI-driven medical diagnostic applications include:
- Medical Imaging Analysis: AI algorithms are used to interpret radiological images such as X-rays, CT scans, and MRIs to identify abnormalities, such as tumors, fractures, or signs of diseases like pneumonia and tuberculosis.
- Genomic Data Analysis: AI can analyze DNA sequencing data, helping to identify genetic predispositions to certain diseases or recommending personalized treatment plans based on genetic information.
- Clinical Decision Support: AI tools provide healthcare providers with evidence-based recommendations for treatment options, aiding doctors in making informed decisions.
- Predictive Analytics: AI can assess patient data to predict future health events, such as the likelihood of heart attacks or strokes, helping in preventative care and early interventions.
- Natural Language Processing (NLP): AI-powered NLP tools assist in processing and analyzing patient records, clinical notes, and medical literature to identify relevant information for diagnosis and treatment.
Accuracy of AI in Medical Diagnosis
AI has demonstrated impressive accuracy in various diagnostic fields, often surpassing human performance in specific tasks. However, there are limitations and challenges to consider when evaluating the role of AI in medical diagnostics.
1. AI in Medical Imaging
AI systems, especially those using deep learning algorithms, have shown considerable success in interpreting medical images. For instance, AI-driven tools have been developed to detect early-stage cancers, including breast cancer, lung cancer, and skin cancers. These systems analyze medical images at a level of detail that often goes unnoticed by human eyes.
In studies, AI systems have achieved accuracy rates comparable to, or even better than, human radiologists in certain image-based diagnoses. For example, AI systems trained to analyze mammograms have demonstrated accuracy levels of over 90%, matching or exceeding the diagnostic performance of radiologists in identifying breast cancer. In some instances, AI tools can also flag images for abnormalities more efficiently, reducing the risk of human error or fatigue.
Despite these successes, AI still faces challenges in medical imaging, particularly in generalization. AI models trained on data from one population may struggle when applied to another population with different demographics, environmental factors, or diseases. Variability in imaging techniques and quality can also affect AI’s accuracy. For example, slight differences in how an image is captured, such as exposure levels or the positioning of the patient, could lead to errors.
2. AI in Predictive Analytics
AI has also shown promise in predicting patient outcomes, such as the likelihood of diseases like diabetes, heart disease, and stroke. By analyzing historical patient data, AI models can detect subtle trends and correlations that might not be immediately evident to human doctors.
For instance, predictive models have been used to anticipate the risk of heart attack by evaluating a patient’s electrocardiogram (ECG) data, lab results, and lifestyle factors. In some cases, these AI tools have helped identify patients at high risk, allowing for early intervention and preventative care.
However, the accuracy of predictive analytics is dependent on the quality and quantity of the data being used. While AI can be very accurate when trained on high-quality, comprehensive datasets, the system’s performance may degrade if the input data is incomplete, outdated, or biased. Additionally, predictions made by AI tools must be considered alongside other clinical factors, such as a patient’s medical history and physician’s knowledge, to ensure the most effective course of action.
3. AI in Genomic Medicine
In the field of genomics, AI has the potential to revolutionize personalized medicine by providing insights into the genetic basis of diseases. AI can help identify genetic mutations associated with conditions like cystic fibrosis, sickle cell anemia, and various cancers. By analyzing vast amounts of genomic data, AI systems can predict the likelihood of genetic disorders and recommend personalized treatment regimens.
AI’s ability to analyze big data has proven useful in interpreting the complexities of human genetics. For instance, AI systems can identify gene expression patterns linked to certain diseases, leading to the development of targeted therapies. While AI has made great strides in this area, understanding the full range of genetic interactions is still a work in progress. Furthermore, the integration of genomic data into clinical practice is complicated by ethical and privacy concerns related to genetic information.
4. AI in Decision Support Systems
AI has been integrated into clinical decision support tools, helping doctors make more informed decisions based on evidence-based guidelines, patient data, and medical literature. These tools can quickly sift through enormous amounts of medical data to provide actionable insights and treatment recommendations.
For example, AI-driven systems like IBM Watson for Oncology analyze vast datasets of clinical trials, medical journals, and patient records to assist doctors in determining the most appropriate cancer treatments. While AI can provide accurate recommendations, its role is limited to augmenting, not replacing, human judgment. Medical professionals must still interpret and apply AI suggestions in the context of the patient’s unique situation.
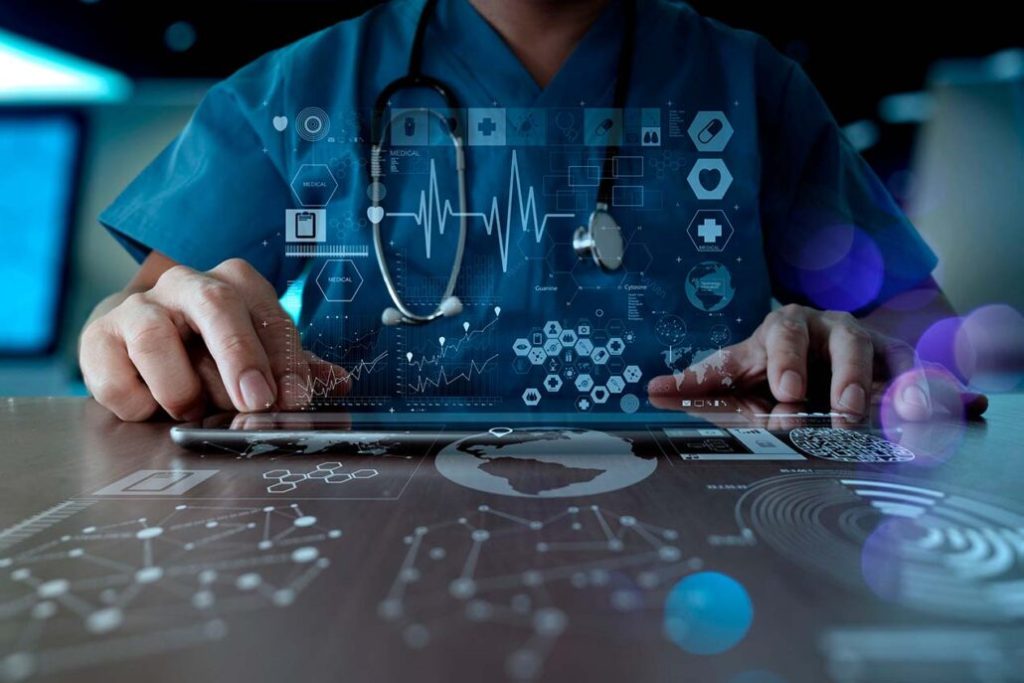
Can AI Replace Human Doctors’ Judgment?
Despite AI’s impressive diagnostic accuracy, it is unlikely that AI will fully replace human doctors’ judgment in the foreseeable future. There are several reasons why human expertise remains essential in healthcare decision-making:
1. Context and Clinical Expertise
AI systems excel at processing large volumes of data and identifying patterns within that data, but they lack the ability to fully understand the broader context of a patient’s condition. Human doctors are trained to consider not only the data but also the patient’s unique life circumstances, emotions, social determinants of health, and personal preferences. These factors are crucial when making decisions about diagnosis and treatment.
For example, in cases of mental health disorders or chronic illness, a physician’s judgment and empathy are essential for understanding the patient’s subjective experience. AI tools can assist in these areas, but they cannot replace the human touch that is vital to effective healthcare.
2. Ethical and Emotional Considerations
Medicine is not only a science but also an art that involves significant ethical decision-making. In complex cases, doctors must navigate difficult decisions that weigh the potential benefits of treatment against risks, costs, and quality of life. Additionally, doctors play a crucial role in communicating sensitive diagnoses and providing emotional support to patients and their families.
AI, by contrast, lacks the emotional intelligence and ethical reasoning required for these nuanced decisions. Although AI can support the decision-making process, it cannot replace the compassionate, empathetic aspects of healthcare that are central to the doctor-patient relationship.
3. The Risk of Over-Reliance on AI
One concern regarding the widespread adoption of AI in medical diagnostics is the risk of over-reliance on technology. AI models, while powerful, are not infallible. Errors in data, model misinterpretations, or biases in the algorithm can lead to inaccurate diagnoses or recommendations.
Human doctors can act as a safeguard against these risks by critically evaluating AI-generated suggestions and considering other factors that the AI might not have accounted for. In particular, healthcare professionals must be vigilant in recognizing when an AI tool may not be performing optimally, such as in cases with incomplete data or atypical patient conditions.
Conclusion: The Future of AI in Medicine
AI has undeniably transformed the healthcare landscape, providing powerful tools to assist in medical diagnosis, improve treatment outcomes, and streamline workflows. However, AI is unlikely to completely replace human doctors’ judgment. Instead, AI should be viewed as a valuable tool that augments and supports the work of healthcare professionals.
The future of AI in healthcare will likely involve collaborative decision-making, where AI assists doctors by providing data-driven insights and predictions, while human doctors apply their clinical expertise, ethical considerations, and empathetic judgment. This synergy between AI and human expertise will enable more efficient, accurate, and personalized care for patients, but the critical role of human professionals in the decision-making process will remain indispensable.
Ultimately, the success of AI in healthcare will depend on its integration into clinical practice in a way that complements human capabilities, rather than replacing them entirely.
Discussion about this post