Introduction: The Intersection of AI and Neuroscience
In the world of scientific research, the boundaries between disciplines are becoming increasingly blurred. The advent of interdisciplinary laboratories, where researchers from diverse fields collaborate, has opened up new avenues for exploration and innovation. One of the most exciting developments in recent years is the fusion of artificial intelligence (AI) and neuroscience. By combining the computational power and advanced algorithms of AI with the study of the brain and nervous system, scientists are embarking on a new era of research that could fundamentally change our understanding of the human brain and revolutionize traditional scientific methodologies.
The marriage of AI and neuroscience is still in its early stages, but it is already showing immense potential. AI’s ability to process vast amounts of data and learn from patterns makes it an ideal tool for tackling the complex, data-rich challenges of neuroscience. Conversely, insights into how the brain functions could help enhance AI algorithms, potentially leading to smarter, more intuitive systems. The question now is: Can this interdisciplinary approach disrupt traditional research models and lead to breakthroughs that were once thought to be beyond our reach?
This article will explore the role of AI in modern neuroscience, examine the rise of interdisciplinary laboratories, and discuss how the synergy between these fields could transform scientific research and innovation.
The Traditional Research Model: A Fragmented Approach
Historically, scientific research has been divided into distinct disciplines, each with its own methodologies, tools, and practices. Neuroscience, for example, has traditionally relied on biological experiments, neuroimaging techniques, and animal models to explore brain function. Similarly, AI has developed primarily within the realm of computer science, with a focus on algorithms, neural networks, and data processing. Although both fields aim to solve complex problems, their methods and approaches have often been siloed, leading to fragmented research and slower progress.
In traditional neuroscience research, a great deal of effort has been dedicated to understanding the mechanisms of the brain, such as how neurons communicate, how different brain regions are activated during various tasks, and how diseases like Alzheimer’s or Parkinson’s impact brain function. However, the vast complexity of the brain—an organ with approximately 86 billion neurons—makes it challenging to draw clear conclusions or even analyze the sheer volume of data involved.
Similarly, in the field of AI, much of the research has been focused on improving algorithms to mimic certain aspects of human cognition, such as learning, pattern recognition, and decision-making. However, these models often lack the complexity and adaptability of the human brain, leading to a gap between biological intelligence and artificial intelligence.
The traditional approach of working in isolated disciplines, though successful in certain areas, has proven to be inadequate when trying to tackle problems as multifaceted and interconnected as those found in neuroscience and AI. This has led to the emergence of interdisciplinary research labs, where scientists from various fields work together to bridge the gap between disciplines.
The Rise of Interdisciplinary Laboratories
The emergence of interdisciplinary laboratories is a direct response to the increasing complexity of modern scientific problems. By pooling expertise from multiple fields, these labs are able to tackle problems from different angles, leading to a more holistic understanding of the issues at hand. In the case of AI and neuroscience, interdisciplinary collaboration has become a natural next step. Neuroscientists bring their knowledge of brain structures and functions, while AI researchers contribute their expertise in machine learning, data analysis, and computational modeling.
These interdisciplinary labs are transforming how research is conducted, offering several advantages over traditional, siloed approaches:
1. Data Integration and Analysis
One of the biggest challenges in neuroscience is managing and interpreting the vast amounts of data generated through neuroimaging, electrophysiology, and other brain research techniques. AI, particularly machine learning, is well-suited to process large datasets and identify patterns that might otherwise go unnoticed. Deep learning algorithms can analyze neuroimaging data to reveal subtle changes in brain structure or function that are indicative of diseases or developmental issues.
By integrating AI with neuroscience, researchers can automate much of the data analysis process, drastically reducing the time needed to draw conclusions. Additionally, AI can help to identify new relationships between different brain regions or uncover hidden patterns in behavior, providing new insights into brain function and cognition.
2. Simulating Brain Activity
One of the most ambitious goals of combining AI with neuroscience is the development of brain simulations. By using AI algorithms to model the complex neural networks of the brain, researchers hope to create accurate simulations of brain activity. These simulations could help us better understand the brain’s inner workings, including how it processes information, stores memories, and makes decisions.
Neuromorphic computing is an area of research focused on building AI systems that mimic the brain’s architecture. This could lead to more efficient, energy-saving AI systems and provide insights into how natural intelligence works. AI-powered brain simulations might also provide a testing ground for new treatments for neurological disorders, allowing researchers to experiment with interventions before applying them to human patients.
3. Cross-Disciplinary Innovation
Interdisciplinary collaboration encourages cross-pollination of ideas. Neuroscientists may find inspiration from AI models to rethink how they approach brain research, while AI researchers may use neuroscience insights to develop new algorithms or architectures. This cross-disciplinary innovation can lead to breakthrough discoveries that would not have been possible within the confines of a single discipline.
For instance, researchers studying cognitive functions like decision-making and memory have drawn inspiration from neuroscience to build reinforcement learning algorithms that mimic the way the brain reinforces certain behaviors. Similarly, advances in neural networks used in AI are increasingly being informed by the structure and function of biological neural networks in the brain.
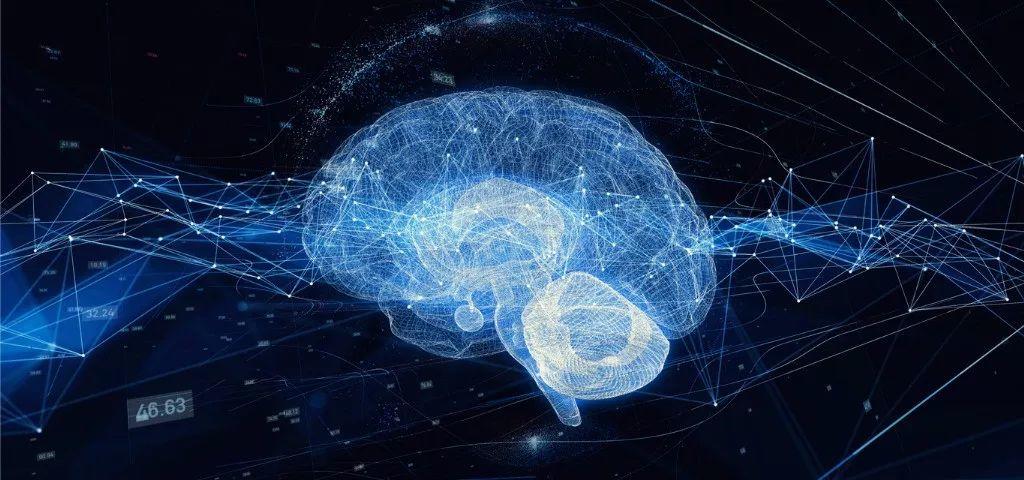
AI and Neuroscience: Disrupting Traditional Research Models
As AI and neuroscience continue to converge, the traditional models of scientific research are being upended. Here are several ways this partnership could disrupt traditional research approaches:
1. Accelerating Scientific Discovery
AI has the potential to accelerate scientific discovery by processing data more quickly and identifying patterns at a scale that is beyond human capacity. Traditional neuroscience often involves labor-intensive methods, such as manual data analysis and hypothesis testing. AI can automate many of these processes, allowing researchers to test hypotheses more efficiently and generate results faster.
This acceleration could lead to quicker breakthroughs in areas like understanding brain diseases, improving mental health treatments, and developing new AI technologies that better mimic human cognition.
2. Revolutionizing Research Methodologies
The integration of AI into neuroscience is revolutionizing research methodologies. Traditional approaches in neuroscience often rely on hypothesis-driven research, where scientists develop theories about brain function and test them through experiments. AI, on the other hand, is more data-driven, often identifying patterns and relationships that researchers might not have considered. By combining these approaches, interdisciplinary labs can take a more holistic, data-driven approach to understanding the brain, allowing for the discovery of new avenues of research.
3. Ethical Implications and New Challenges
While the convergence of AI and neuroscience presents numerous opportunities, it also introduces new ethical and societal challenges. For instance, AI models trained on brain data could potentially raise privacy concerns, as these models may have the ability to decode or infer sensitive information about an individual’s thoughts or behaviors. Furthermore, the development of advanced brain-computer interfaces could raise questions about neuroethics and the potential for mind manipulation.
As AI becomes more deeply integrated with neuroscience, researchers must also consider the ethical implications of their work. Ensuring that these technologies are used responsibly and transparently will be crucial to their success and acceptance.
Conclusion: A New Era of Research and Innovation
The rise of interdisciplinary laboratories combining AI and neuroscience is set to reshape the landscape of scientific research. By integrating AI’s computational power with the biological insights of neuroscience, researchers can tackle some of the most challenging questions about the brain and cognition. This convergence has the potential to accelerate discoveries, transform research methodologies, and provide new solutions for treating neurological disorders.
While there are still challenges and ethical considerations to address, the potential for AI and neuroscience to disrupt traditional research models is undeniable. As these two fields continue to evolve together, they may unlock insights that were once thought to be beyond our grasp, leading to groundbreaking advances in both neuroscience and artificial intelligence.
Discussion about this post