Introduction
Artificial intelligence (AI) is rapidly advancing, and its integration into various industries—from healthcare and finance to transportation and entertainment—promises to reshape the future in profound ways. With AI’s ability to process vast amounts of data, make decisions, and even perform tasks traditionally carried out by humans, we are witnessing the rise of intelligent systems that can learn, adapt, and, in some cases, surpass human performance.
While AI holds enormous potential, it also brings with it a range of ethical concerns that are becoming increasingly difficult to ignore. As AI systems become more autonomous and capable, the questions about their morality, accountability, fairness, and the impact they will have on society grow more urgent. For instance, who is responsible when an AI makes a harmful decision? Can an AI system be truly unbiased? What happens when AI systems make decisions that affect human lives, such as in healthcare, criminal justice, or finance?
This article will explore the ethical dilemmas associated with AI, examining issues such as bias, accountability, privacy, and the implications for employment and human rights. Furthermore, it will discuss potential solutions and frameworks that can help address these challenges, ensuring that AI is developed and used in ways that benefit humanity as a whole.
1. The Rise of Artificial Intelligence: Opportunities and Concerns
A. AI’s Role in Society
AI is revolutionizing industries and everyday life. It is integrated into autonomous vehicles, healthcare diagnostics, financial advising, and even entertainment recommendations. AI systems can learn from data, improving their performance over time, and in some cases, outperform humans in specific tasks. However, this rapid advancement raises important ethical concerns.
B. Defining Ethical Issues in AI
Ethics in AI concerns how AI systems should behave in a way that aligns with human values and norms. While technology often develops faster than societal understanding, AI introduces new challenges to ethics, as these systems can potentially make autonomous decisions without human intervention.
2. Ethical Challenges Posed by AI
A. Bias and Discrimination
AI systems learn from historical data, and if this data is biased or incomplete, the AI can inherit and even amplify these biases. In critical areas like hiring, law enforcement, and healthcare, biased AI systems can perpetuate existing societal inequalities.
- Hiring and Employment: AI systems designed to screen job applicants could unintentionally favor certain demographics over others based on historical data that reflects past biases. For example, an AI trained on data from predominantly male-dominated industries could unintentionally prioritize male applicants over female ones.
- Criminal Justice: AI-based predictive policing and sentencing tools have raised concerns about reinforcing racial biases. Systems that predict recidivism or determine parole eligibility may rely on biased historical data, leading to unfair treatment of minority groups.
- Healthcare: AI systems used in diagnostics could misinterpret data from underrepresented groups, leading to misdiagnoses or unequal access to care. For example, an AI model trained primarily on data from Caucasian patients may not be as effective at diagnosing conditions in people of color.
B. Accountability and Responsibility
When AI systems make decisions—especially in critical areas like healthcare, finance, or autonomous vehicles—the question of accountability becomes complex. If an AI makes a mistake or causes harm, who is responsible?
- Liability: Is the creator of the AI responsible for its actions, or is the user of the AI system at fault? In cases where an autonomous vehicle crashes, should the company that designed the AI be held accountable, or is the responsibility on the owner or operator of the vehicle?
- Transparency: Many AI systems, particularly those based on deep learning, function as “black boxes,” meaning that it is difficult to understand how they arrive at certain decisions. This lack of transparency makes it challenging to hold systems accountable, as their decision-making process is not always explainable or accessible.
C. Privacy and Surveillance
AI systems have the ability to collect, analyze, and store vast amounts of personal data, raising concerns about privacy violations. For example, facial recognition technology can track individuals in public spaces without their consent, leading to potential surveillance issues.
- Surveillance: In authoritarian regimes, AI-powered surveillance systems may be used to monitor and control citizens, infringing on their privacy and freedom. On the other hand, in democratic societies, AI surveillance tools can help monitor public spaces for security reasons but may also infringe upon individuals’ rights to privacy.
- Data Security: AI relies on vast amounts of personal data to function effectively, and this data could be vulnerable to breaches or misuse. A healthcare AI that stores patient data, for instance, could be a prime target for cyberattacks, potentially compromising sensitive medical information.
D. Job Displacement and Economic Inequality
The increasing use of AI in the workforce raises concerns about job displacement. As AI systems take over tasks traditionally performed by humans, there is a risk that large segments of the workforce may become obsolete, leading to economic inequality.
- Automation and Employment: AI systems are already being used to automate tasks in industries like manufacturing, transportation, and retail. While this increases efficiency, it also reduces the need for human workers, particularly in routine and low-skilled jobs.
- Wealth Distribution: The benefits of AI may disproportionately accrue to those who own or control AI technologies, creating a wealth gap between tech companies and workers. Governments may need to implement policies like universal basic income (UBI) or retraining programs to ensure equitable distribution of wealth and opportunities in an AI-driven economy.
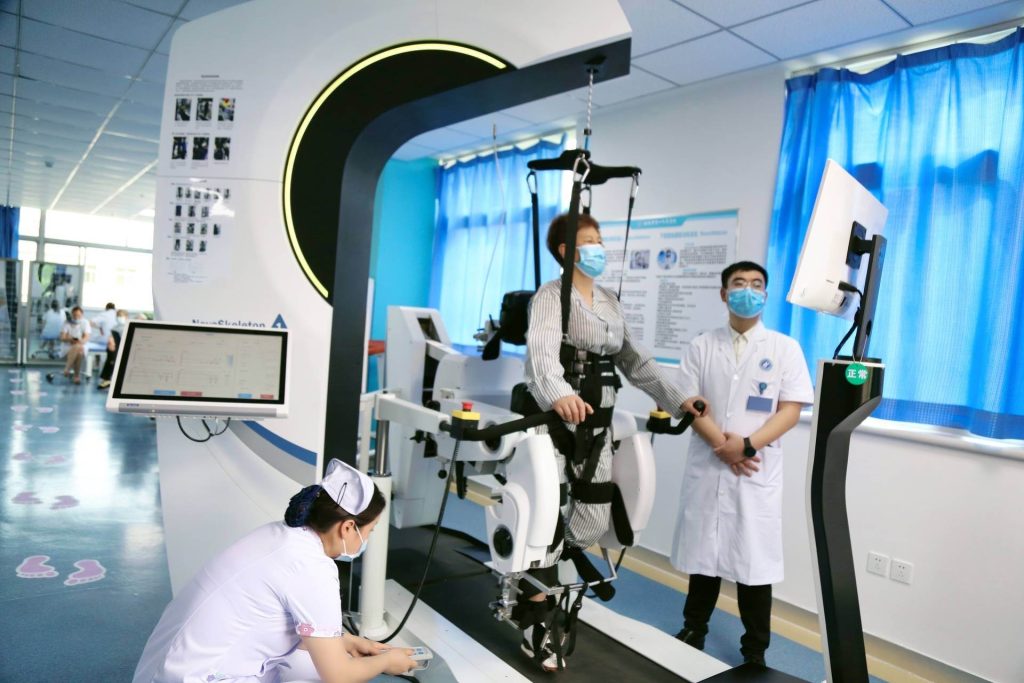
3. How to Address Ethical Issues in AI
A. Ensuring Fairness and Reducing Bias
To combat the potential for bias in AI systems, developers must take proactive steps to ensure that their models are trained on diverse and representative datasets. This can help prevent the amplification of existing societal biases and ensure that AI systems work fairly for all demographics.
- Diverse Data: AI models should be trained on data that represents all relevant demographic groups, including gender, race, and socioeconomic status. This helps to ensure that the AI performs accurately and fairly across different groups.
- Bias Detection and Mitigation: Regular auditing of AI systems is essential to detect and mitigate bias. Machine learning engineers and data scientists can employ techniques such as fairness-aware algorithms and bias correction methods to improve the performance of AI systems.
B. Establishing Clear Accountability Structures
As AI systems become more autonomous, it is critical to establish clear accountability structures to determine who is responsible for the decisions made by AI systems.
- Regulation and Governance: Governments and international bodies must create clear regulations that outline who is responsible for AI-related harm, whether it be the developers, users, or manufacturers. Legal frameworks should be designed to address liability and transparency in AI systems.
- Explainability: Developers must prioritize the creation of AI models that are explainable. This allows both users and regulators to understand how decisions are made, which improves accountability and helps build trust in AI systems.
C. Protecting Privacy and Data Security
To ensure privacy in an AI-driven world, it is necessary to create regulations that govern the use of personal data. Data protection laws like the General Data Protection Regulation (GDPR) in the European Union provide a useful model for how data privacy can be protected.
- Data Anonymization: AI systems should be designed to anonymize personal data to protect individuals’ privacy. This involves removing any personally identifiable information from the datasets that AI models use, reducing the risk of misuse or exposure.
- User Consent: AI systems should operate on the principle of informed consent. Users should be clearly informed about what data is being collected and how it will be used, with the option to opt-out.
D. Supporting Workforce Transition and Addressing Economic Inequality
To address the potential for job displacement, governments and organizations need to invest in retraining and reskilling workers to prepare them for the future job market. Public policies must support the transition of workers into new roles and ensure that the benefits of AI are widely shared.
- Retraining Programs: Governments and private companies can collaborate to provide retraining programs for workers whose jobs are at risk of being automated. These programs should focus on high-demand skills such as AI, data science, and digital literacy.
- Universal Basic Income (UBI): Some experts argue that UBI could help mitigate the economic disruptions caused by AI and automation. By providing a basic income to all citizens, UBI could help ensure that people continue to have a stable source of income even as AI takes over certain jobs.
4. Conclusion: Moving Forward with Ethical AI
As AI continues to evolve, addressing its ethical challenges will be crucial to ensuring that it benefits society as a whole. By proactively addressing issues such as bias, accountability, privacy, and job displacement, we can ensure that AI becomes a tool for improving lives rather than perpetuating harm. Developing clear ethical guidelines, investing in fairness, and establishing transparent AI governance will help to build a future where AI works for everyone.
Ultimately, the success of AI in a moral and just society will depend on the collective efforts of governments, developers, and citizens to navigate these ethical challenges. With careful planning, AI can be harnessed to solve pressing global challenges, from healthcare and climate change to education and economic inequality.
Discussion about this post