Introduction
Artificial intelligence (AI) has revolutionized numerous sectors in recent years, from healthcare and finance to entertainment and transportation. Its impact, however, is perhaps most profound in the field of scientific research, where it has the potential to accelerate discoveries, solve complex problems, and revolutionize entire industries. In an era where innovation and research are critical to solving some of the world’s most pressing challenges—such as climate change, pandemics, and sustainability—the question arises: Can AI help drive breakthrough discoveries in scientific research?
AI’s ability to process vast amounts of data, identify patterns, and make predictions far beyond the capabilities of human researchers has made it an invaluable tool in various areas of scientific inquiry. Whether it’s through speeding up drug discovery, simulating complex molecular interactions, optimizing experimental designs, or even analyzing astronomical data, AI is poised to play an instrumental role in reshaping the future of scientific research.
This article explores the ways in which AI is already transforming the research landscape, the potential it holds for accelerating breakthroughs, and the challenges and limitations that still need to be addressed for AI to fully realize its potential in research.
1. AI in Drug Discovery and Healthcare Research
A. Accelerating Drug Development
One of the most exciting areas where AI is already making a significant impact is in drug discovery. Traditionally, drug development has been a long, expensive, and often inefficient process, taking years or even decades to bring a new drug to market. However, AI is changing this paradigm by dramatically speeding up the process and making it more cost-effective.
- Predicting Molecular Interactions: AI algorithms, particularly deep learning models, can analyze vast chemical libraries and predict how different molecules might interact with one another. By identifying potential drug candidates and predicting their effectiveness before conducting expensive and time-consuming laboratory experiments, AI reduces the trial-and-error approach traditionally used in drug discovery.
- AI and Precision Medicine: AI is also playing a significant role in the development of precision medicine, where treatments are tailored to the genetic makeup of individual patients. Machine learning models can analyze large datasets of genetic information, patient histories, and clinical trial results to predict which therapies are most likely to work for specific patients, thereby accelerating the discovery of personalized treatments for diseases like cancer, diabetes, and rare genetic disorders.
- Example: AlphaFold and Protein Folding: One of the most remarkable AI-driven breakthroughs in recent years was made by DeepMind’s AlphaFold, which accurately predicted the 3D structures of proteins. Protein folding, a complex process central to understanding biological functions, had remained a major challenge in biology for decades. AlphaFold’s AI model has already contributed to advancing our understanding of diseases like Alzheimer’s, cancer, and COVID-19, and it holds the promise of significantly accelerating drug discovery.
B. Speeding Up Clinical Trials
Another way AI is helping accelerate scientific breakthroughs in healthcare is through the optimization of clinical trials. Clinical trials are essential for testing new drugs, therapies, and medical devices, but they often take years to complete and are fraught with challenges related to patient recruitment, data analysis, and trial design.
- Patient Recruitment and Data Integration: AI algorithms can sift through electronic health records (EHRs) to identify suitable candidates for clinical trials, ensuring a more diverse and representative pool of participants. Additionally, AI can integrate and analyze data from different sources (such as genetic data, medical imaging, and lab results) to help researchers identify the most promising treatment pathways more quickly.
- Real-Time Monitoring and Predictive Analytics: AI models can track patient responses in real-time, enabling faster adjustments to treatment protocols and better management of trial outcomes. By leveraging predictive analytics, AI can help researchers identify potential issues earlier in the process, reducing the risk of failure and increasing the likelihood of success in clinical trials.
2. AI in Environmental Research and Climate Science
A. Addressing Climate Change
Climate change is perhaps the most urgent global challenge of our time, and AI is proving to be an invaluable tool in both understanding and combating its effects. AI-driven research can accelerate the identification of climate solutions, improve the prediction of climate patterns, and optimize environmental monitoring systems.
- Predictive Modeling and Climate Forecasting: AI is helping to develop more accurate climate models that can predict the impact of climate change on various regions and ecosystems. Machine learning algorithms are capable of analyzing vast amounts of data from climate sensors, satellites, and historical records to make more accurate predictions about temperature changes, extreme weather events, and sea level rise.
- AI in Renewable Energy Optimization: AI is being used to optimize renewable energy systems, such as wind and solar farms, by predicting energy production patterns and adjusting systems for maximum efficiency. For example, AI-powered predictive maintenance systems can identify potential issues in wind turbines or solar panels before they cause major breakdowns, reducing downtime and increasing energy output.
- AI for Conservation and Biodiversity Monitoring: AI is also being used to monitor biodiversity and protect endangered species. Computer vision systems, for example, can analyze camera trap images to identify and track wildlife, providing researchers with valuable data on animal populations and behavior without the need for human intervention.
B. Sustainable Agriculture and Resource Management
AI is revolutionizing agricultural research by helping farmers optimize resource use, improve crop yields, and reduce environmental impacts. The application of AI in agriculture has the potential to address food security challenges while promoting more sustainable farming practices.
- Precision Agriculture: AI-powered drones and sensors are used in precision agriculture to monitor soil health, crop growth, and irrigation needs. This enables farmers to make data-driven decisions on when and where to apply fertilizers, pesticides, and water, thus reducing waste and minimizing the environmental impact of farming.
- Climate-Resilient Crops: Machine learning algorithms are also being used to identify genes responsible for drought resistance, pest resistance, and improved nutritional value in crops. This research is helping to develop climate-resilient crops that can withstand extreme weather events and changing climate conditions, ensuring food security in the face of global climate change.
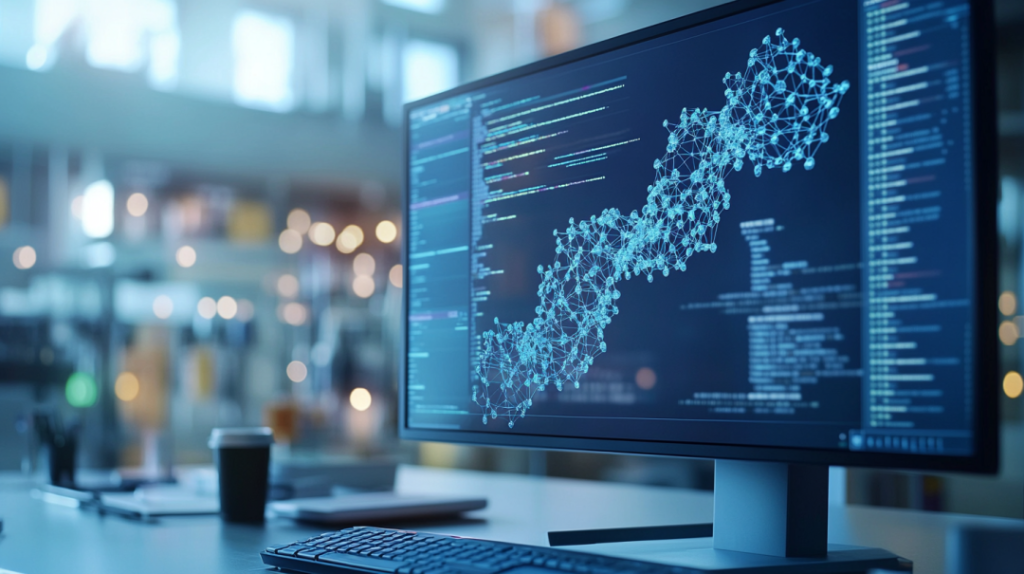
3. AI in Fundamental Scientific Research
A. AI in Physics and Materials Science
AI is accelerating discoveries in fields such as physics and materials science, where large-scale data analysis and complex simulations are often required. Researchers are using AI to model physical systems, identify new materials with unique properties, and explore phenomena that are difficult to observe directly.
- Materials Discovery: AI is being used to discover new materials with properties that could revolutionize industries, from superconductors to energy storage materials. Machine learning algorithms can analyze vast databases of known materials to predict the properties of new compounds, significantly speeding up the process of materials discovery.
- Quantum Computing: Quantum computing represents a frontier in scientific research, and AI is playing a role in solving some of the challenges associated with quantum computing. AI can be used to optimize quantum algorithms, manage error correction, and enhance the performance of quantum hardware, potentially unlocking breakthroughs in fields like cryptography, drug discovery, and complex system simulations.
B. AI in Astrophysics and Astronomy
In the field of astrophysics, AI is playing an increasingly important role in analyzing data from telescopes and satellites. The sheer volume of data produced by astronomical instruments makes it difficult for human researchers to manually analyze, but AI can process this data much faster and more efficiently.
- Identifying Exoplanets: AI has been instrumental in identifying exoplanets (planets outside our solar system) by analyzing data from space telescopes like Kepler. Machine learning algorithms are used to detect small dips in starlight caused by planets passing in front of their stars, a process known as the transit method. AI has enabled the discovery of thousands of exoplanets, many of which were previously undetectable using traditional methods.
- Astronomical Data Processing: AI is also being used to process vast amounts of data from radio telescopes and other observational instruments. For example, AI algorithms are used to identify patterns in the cosmic microwave background radiation, helping researchers understand the early history of the universe.
4. Challenges and Limitations
While AI holds immense potential to accelerate breakthroughs in research, several challenges must be overcome to maximize its impact.
- Data Quality and Bias: AI is heavily reliant on data, and the quality of the data used in training models is crucial. If the data is biased or incomplete, AI models may produce inaccurate or misleading results. In scientific research, this is particularly problematic because even small errors in data analysis can lead to flawed conclusions.
- Interdisciplinary Collaboration: The use of AI in research requires collaboration between scientists, engineers, and AI experts. This interdisciplinary approach can sometimes be challenging, as researchers in different fields may have different priorities, methodologies, and levels of understanding of AI.
- Ethical and Privacy Concerns: In some areas of research, particularly in healthcare and genomics, AI-driven analysis raises ethical and privacy concerns. Protecting sensitive data, ensuring transparency in AI algorithms, and addressing potential biases are critical issues that need to be addressed as AI becomes more integrated into scientific research.
5. Conclusion: A New Era of Scientific Discovery
AI has the potential to dramatically accelerate the pace of scientific discoveries by enabling researchers to process vast amounts of data, simulate complex systems, and identify patterns that would otherwise go unnoticed. From healthcare and environmental research to fundamental scientific inquiry, AI is already driving breakthroughs in numerous fields.
However, realizing the full potential of AI in research requires overcoming challenges related to data quality, interdisciplinary collaboration, and ethical considerations. As AI technology continues to evolve, its role in accelerating scientific discovery will only grow, ushering in a new era of innovation that could help solve some of the world’s most pressing challenges.
In summary, AI’s application in research is not only accelerating breakthroughs; it is fundamentally changing the way we approach scientific inquiry. With continued advancements in AI, the future of research looks poised to be more dynamic, efficient, and impactful than ever before.
Discussion about this post