Artificial Intelligence’s Leap in Mathematical Acumen at the IMO
DeepMind’s AlphaProof and AlphaGeo have procured a feat of resonant echoes bearing resemblance to pivotal moments such as Deep Blue’s victory over Kasparov and AlphaGo’s win against Lee Sedol, marking a significant milestone in the saga of artificial intelligence meeting human intellect at its zenith. These AI prodigies have unprecedently solved four out of six problems at the International Mathematical Olympiad, securing a silver medal. This landmark achievement not only joins the ranks of AI’s pinnacles of success but ignites anew the discourse on the boundaries of machine intelligence.
The significance of early detection and nurturing of mathematical prodigies has garnered widespread recognition. A radical pedagogy takes shape, advocating the transformation of cutting-edge scientific queries into abstract foundational mathematical problems void of the need for specialized knowledge, seeking to scout research talents from tender ages. In this spirit, intricate scientific paradigms such as molecular structure and linear programming are distilled into fundamental mathematical inquiries akin to the well-known ‘chickens and rabbits’ or ‘cows and grass’ puzzles. This approach diverges from traditional knowledge-centric education, examinating ‘fluid intelligence’ which encompasses reasoning and inductive skills. Moreover, the methodology adopted in Olympiad problem-solving aligns with the essence of scientific inquiry: abstracting specific issues or concretizing abstract ones, all the while oscillating across various tiers of abstraction. This innovative approach has garnered success in mining mathematical prowess—several Olympiad contenders progressing to become esteemed mathematicians, with a notable sixteen achieving the prestigious Fields Medal.
The general logical aptitude emphasized in Olympiad problems renders them an idyllic gauge for evaluating the deductive faculties of artificial intelligence. Distinct from tests geared towards specific knowledge domains and elementary logic, the Olympiad presents a challenging array where multiple layers of logical deduction and exhaustive considerations come into play. This calls for universally adaptable reasoning tactics over rote pre-trained solutions—the very essence humans hinge upon for excelling in mathematical Olympiads and conducting research.
AlphaProof/AlphaGeo’s advent to the podium at the International Mathematical Olympiad signifies an evolutionary stride in AI’s capacity for universal reasoning. Differing from Deep Blue and AlphaGo, which outmaneuvered human champions, the Olympiad spans a broader spectrum, demanding higher creative thinking and the capacity to solve unprecedented problems while holding rigor to exacting standards. These attributes foreshadow AI’s potential for supplanting humans in scientific pursuits.
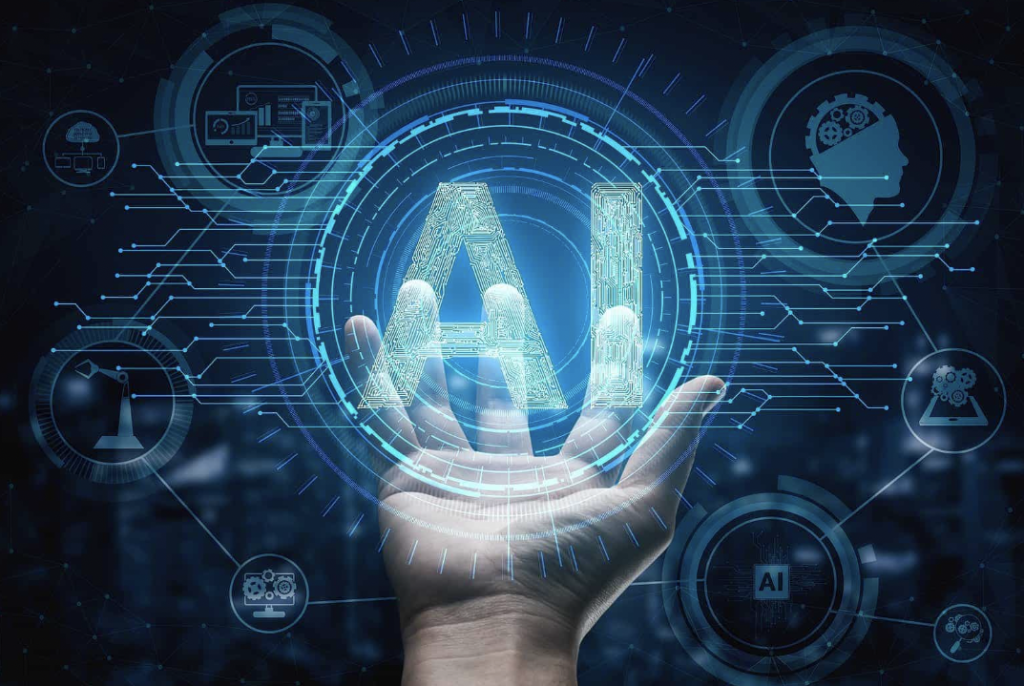
The breakthroughs of AlphaProof/AlphaGeo at the Olympiad emanate from a trifecta of innovations: a neural-symbolic dual-system architecture, synthetic data training, and exploratory analogy-making.
The ingenious neural-symbolic dual-system architecture melds the strengths of neural networks with those of symbolic systems. Neural networks, basing on deep-learning models, are proficient in inductive reasoning, ferreting out latent patterns in vast data streams. While susceptible to “hallucinations,” their “creative” capabilities are invaluable for breaching conventional thought barriers. The symbolic system’s forte, in contrast, is rigid logic deduction, delivering accurate inferences within a logical scaffold. DeepMind’s integration of these systems has yielded a unique problem-solving modality.
The machine proof system garnering widespread mathematical attention is the Lean language, the format employed by the IMO for AI competitors. It commences by translating problems into machine-readable Lean propositions, enabling symbolic systems to derive further propositions through rigorous deduction. If this doesn’t suffice, neural networks assume the mantle, embracing ‘diffusive thinking’ (grounded in Monte Carlo tree searches) to hypothesize intermediary propositions that bridge given conditions and conjectures. Extensive training has honed the neural network’s acumen in pinpointing critical reasoning junctures.
Secondly, the pioneering use of ‘synthetic data’ to fabricate training sets. Symbolic engines generated billions of Olympiad-like problems that, though lacking the subtlety of actual competition challenges, assured accuracy. These problems coerced the neural network and symbolic system into collaborative solutions, emphasizing training on Monte Carlo tree search junctures inspired by the neural network, thus enhancing the model’s capacity to foresee pivotal reasoning phases.
Lastly, the AI’s module for example conjecture and validation stands testament to its novelty. Research published in Nature by DeepMind and collaborator mathematicians detailed the deep-learning model’s potential applications in avant-garde mathematics, with AlphaProof exemplifying the method. Mathematicians operate similarly, juxtaposing ‘explorative diffusion’ with stringent proof methodologies. Initially conjecturing examples, they rigorously validate propositions against them. If inconsistencies arise, they intuitively refine hypotheses, discard errant examples, and continue validation. The symbolic engine crafts and validates examples, while the diffusive neural network refines the hypothesis. If sufficient instances are synthesized, the network discerns more plausible conjectures from emergent patterns—insights drawn from DeepMind’s forays into topology and abstract algebra owe their success to deep learning’s aptitude for unraveling subtle, non-linear, computation-intensive regularities.
Discussion about this post