Blurred Boundaries in the Age of Synthesized Speech
The frontier between AI-generated synthetic voices and authentic human speech grows evermore indistinct in the era of large models, escalating the urgency to enhance recognition technologies that keep pace. On July 23rd, the pinnacle of the 9th Annual Xinya Technology Cup Global AI Algorithm Competition was held in Shanghai, focused on the critical discipline of deep fake voice detection. Contestants were inspired to wield the power of deep learning and adversarial AI to engineer models capable of discerning deceptive auditory content with precision.
Deep fakes represent the ominous advent of utilizing deep learning and artificial intelligence to craft convincingly authentic fabrications. The rise of large models fertilizes the ground for deep fakery—merely by prompt input, AI systems unleash a phantasmagoria of images, videos, and audios that challenge reality.
Take fake voices, for instance: large models usher forth various counterfeit utterances, more life-like, anthropomorphic, and conversational than ever before, escalating the challenge for fake voice recognition. “In some high-stakes scenarios, we encounter AI-generated voice fraud. However, the development of voice authentication lags behind that of voice synthesis technologies,” Chen Lei, the Vice President and Head of Big Data and AI at Xinya Technology, conveyed this concern.
In the heated crucible of the finals, contestants deployed diverse algorithmic models and training methodologies to sniff out fake voices, including tools based on large models and traditional end-to-end techniques. The latter, leaner in parameters, targets specific issues vertically while large models, though parameter-heavy and data-thirsty, exhibit robust generalizability, especially distinguishing those deceits concocted by large models.
Xinya Technology’s algorithm scientist, Lu Qiang, unveiled that the qualifications’ dataset constituted mainly fake voices generated by traditional end-to-end TTS systems, posing a lesser difficulty. However, the semi-finals escalated in challenge with the inclusion of fakes wrought from the latest large models, re-recorded fakes, and hybrids of genuine and spurious vocal samples spanning more than five languages, including English, French, and Spanish. “The semi-finals saw a spike in difficulty with the latest large models blurring lines seamlessly, thus demanding equivalent advancements in deep fake detection techniques.”
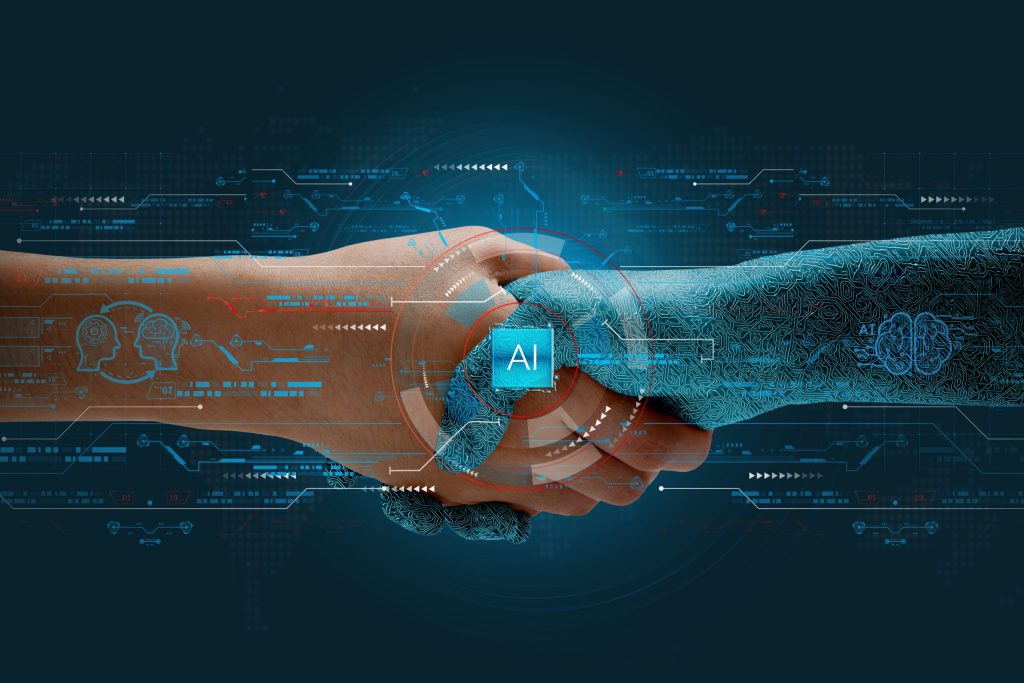
“We strategically injected new scenario data into the competition, like re-recorded fake voices—real voices re-recorded multiple times to create fake data. We regard these as fake voices,” Lu Qiang articulated. Addressing this particular scenario, the contest constructed adversarial data by slicing and amalgamating real and fake voices, eschewing subjectivity from manual listening and tagging, “Any slice of falsehood renders the whole clip fake, more closely mimicking real-world conditions, yet amplifying the recognition challenge. Overcoming re-recordings and real-fake contention holds academic merit.” Lu Qiang also highlighted the instrumental role of text, video, and other multimodal information in voice authentication, with large models and multimodality marking critical future trajectories for voice authentication.
In the “race” between fake-generation technologies and their detection counterparts, both advance in a spiraled ascent. According to Chen Lei, research on large voice models must elevate practical issues to academic questions, which, once resolved academically, can tailor to concrete business needs through engineering. Cross-disciplinary endeavors are essential for developing detection technologies, currently dominated by software algorithms, with a prospective shift towards integration with hardware—aids in tracing voice captures to bolster fake voice risk management from a hardware standpoint.
“The quest for authentication is inexhaustible,” Chen Lei asserted, “As long as generative AI has not reached its terminus, the pursuit of detection will persist unabated.” Post-competition, Xinya Technology plans to open-source the data for broader academic pursuits, share contestants’ anonymized resources for communal learning, and, drawing from the avant-garde model concepts, construct an AIGC authentication platform. He envisions generative AI adhering to governance regulations, with the onus on regulatory frameworks to steer and refine AI governance. He advocates for a coalition in the industry, collectively forging safeguards against systemic risks.
Discussion about this post